All Features
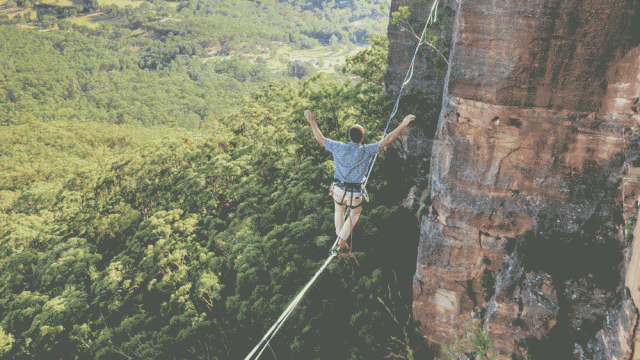
Michael Mills
Risk-based thinking—it sounds easy. How hard can it be to think about risk? But did you know that the phrase “risk-based thinking” was only invented in 2015?
Did you know that the ISO says “risk-based thinking” is one of the foundations of quality management, but never defines it? Or that it…
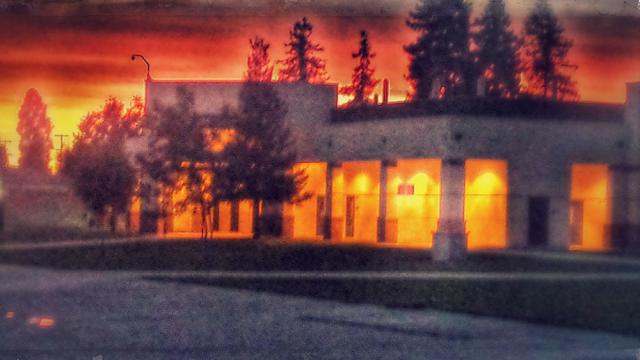
William A. Levinson
The International Accreditation Forum (IAF) and ISO have published a joint communiqué to require organizations to “consider” climate change in the context of risks and opportunities relevant to the management system.
Although this is pursuant to the London Declaration, which has goals for…
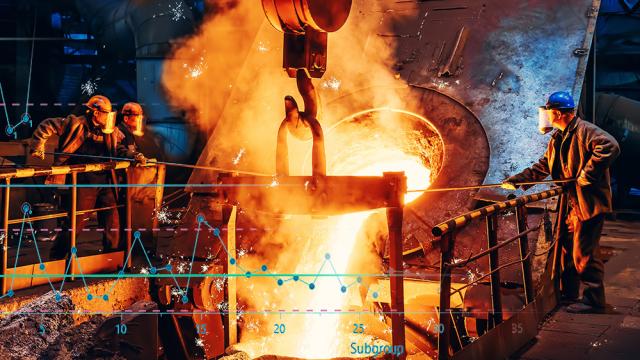
Scott A. Hindle
Walter A. Shewhart is lauded as the Father of Statistical Process Control (SPC) and is perhaps best remembered for the SPC control chart. The first record of Shewhart’s control chart is found in a Bell Telephone Laboratories internal memo from May 16, 1924, making today the 100th anniversary of…
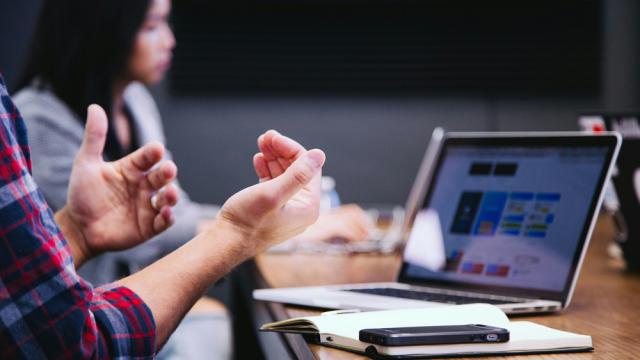
Stephanie Ojeda
An analysis of U.S. Food and Drug Administration (FDA) warning letters by the Food and Drug Law Institute reveals a perhaps not-so-surprising link between training gaps and FDA violations.
It’s one of several factors motivating companies to switch to automated training management software. The…
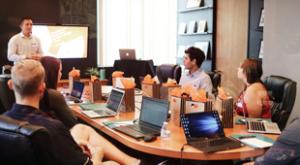
Mark Rosenthal
Continuing on the theme of value stream mapping (and process mapping in general) from my article “Where is your value stream map?”, I outlined the typical scenario: The map is built by the continuous improvement team, and they are the ones primarily engaged in the conversations about how to close…
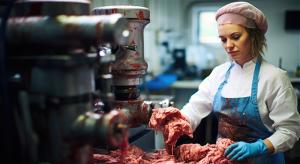
Scott A. Hindle, Douglas C. Fair
So far in this series our focus has remained on statistical process control (SPC) in manufacturing. We’ve alternated between more traditional uses of SPC that remain relevant in this digital era and discussing uses of SPC and its related techniques that are enabled by the marvels of modern…
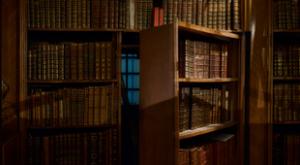
Adam Grant
Nano Tools for Leaders—a collaboration between Wharton Executive Education and Wharton’s Center for Leadership and Change Management—are fast, effective tools that you can learn and start using in less than 15 minutes, with the potential to significantly affect your success and the engagement and…
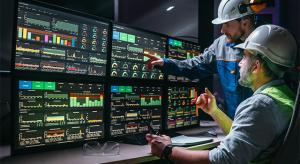
Scott A. Hindle, Douglas C. Fair
You are assigned a new task to demonstrate that an existing process will have the capability to meet newer and tighter specifications. The change in specifications for critical-to-quality characteristic P is due to new regulatory requirements; hence, the specifications must be met. The task is…
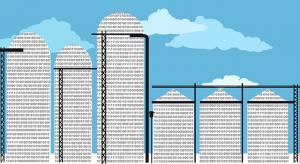
Douglas C. Fair, Scott A. Hindle
Just a few decades ago, today’s personal technology was a science fiction pipe dream. Powerful computers (smart phones) that fit in our pockets; global positioning satellites for our traveling convenience; and homes where lights, security systems, and locks can be controlled remotely. It’s all just…
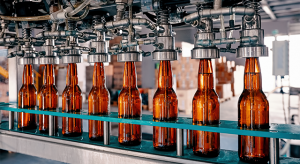
Scott A. Hindle, Douglas C. Fair
Parts 1, 2, and 3 of our series on statistical process control (SPC) have shown how data can be thoughtfully used to enable learning and improvement—and consequently, better product quality and lower production costs. Another area of SPC to tap into is that of measurement methods. How do we ensure…
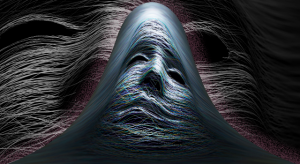
Donald J. Wheeler
Fourteen years ago, I published “Do You Have Leptokurtophobia?” Based on the reaction to that column, the message was needed. In this column, I would like to explain the symptoms of leptokurtophobia and the cure for this pandemic affliction.
Leptokurtosis is a Greek word that literally means “thin…
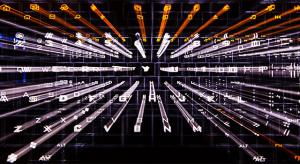
Douglas C. Fair, Scott A. Hindle
Data overload has become a common malady. Modern data collection technologies and low-cost database storage have motivated companies to collect data on almost everything. The result? Data overload. Unfortunately, few companies leverage the information hidden away in those terabytes of data.
There…
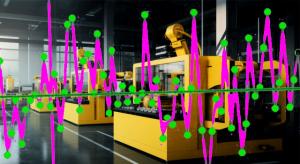
Scott A. Hindle, Douglas C. Fair
We are one year away from the 100th anniversary of the creation of the control chart: Walter Shewhart created the control chart in 1924 as an aid to Western Electric’s manufacturing operations. Since it’s almost prehistoric, is it now time to leave the control chart technique—that started out using…
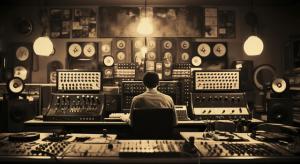
Donald J. Wheeler
In last month’s column, we looked at how process-hyphen-control algorithms work with a process that is subject to occasional upsets. This column will consider how they work with a well-behaved process.
Last month we saw that process adjustments can reduce variation when they are reacting to real…
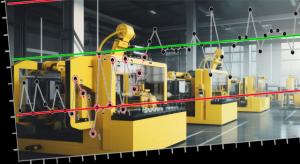
Douglas C. Fair, Scott A. Hindle
Today’s manufacturing systems have become more automated, data-driven, and sophisticated than ever before. Visit any modern shop floor and you’ll find a plethora of IT systems, HMIs, PLC data streams, machine controllers, engineering support, and other digital initiatives, all vying to improve…
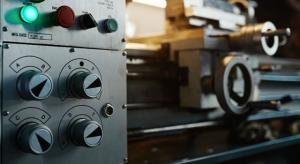
Donald J. Wheeler
Many articles and some textbooks describe process behavior charts as a manual technique for keeping a process on target. For example, in Norway the words used for SPC (statistical process control) translate as “statistical process steering.” Here, we’ll look at using a process behavior chart to…
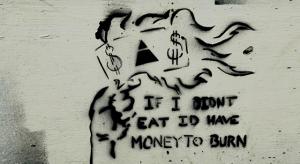
William A. Levinson
Inflation is a serious national issue. Credit agency Fitch Ratings just downgraded the U.S. credit rating—as in the “full faith and credit of the United States”—from AAA to AA+.1 This doubtlessly reflects the fact that our national debt exceeds $31 trillion, or almost $100,000 for every American,…
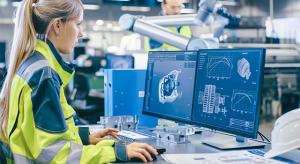
Donald J. Wheeler
As we learned last month, the precision to tolerance ratio is a trigonometric function multiplied by a scalar constant. This means that it should never be interpreted as a proportion or percentage. Yet the simple P/T ratio is being used, and misunderstood, all over the world. So how can we properly…
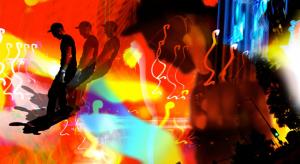
Donald J. Wheeler
The keys to effective process behavior charts are rational sampling and rational subgrouping. As implied by the word rational, we must use our knowledge of the context to collect and organize data in a way that answers the interesting questions. This column will show the role that sample frequency…
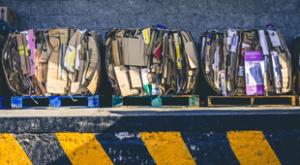
Paul Laughlin
Continuing our thinking about ways for data leaders to save money during a recession, this article drills into saving on your data usage. Following my last post reminiscing on the lessons I learned during past recessions, the early environmentalist slogan “reduce, reuse, recycle” has stayed in my…
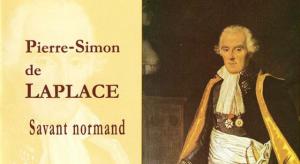
Donald J. Wheeler
As the foundations of modern science were being laid, the need for a model for the uncertainty in a measurement became apparent. Here we look at the development of the theory of measurement error and discover its consequences.
The problem may be expressed as follows: Repeated measurements of one…
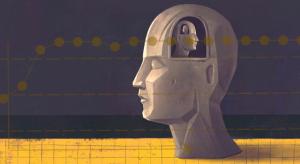
Donald J. Wheeler, Al Pfadt
In memory of Al Phadt, Ph.D.
This article is a reprint of a paper Al and I presented several years ago. It illustrates how the interpretation and visual display of data in their context can facilitate discovery. Al’s integrated approach is a classic example not only for clinical practitioners but…
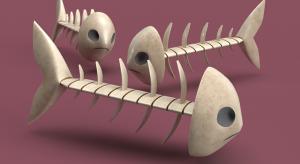
Alan Metzel
Almost seven years ago, Quality Digest presented a short article by Matthew Barsalou titled “A Worksheet for Ishikawa Diagrams.” At the time, I commented concerning enhancements that provide greater granularity. Indicating that he would probably have little time to devote to such a project,…
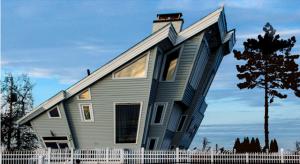
Donald J. Wheeler
The computation for skewness does not fully describe everything that happens as a distribution becomes more skewed. Here we shall use some examples to visualize just what skewness does—and does not—involve.
The mean for a probability model describes the balance point. The standard deviation…
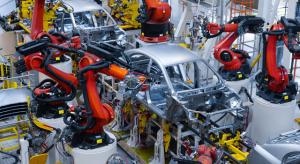
William A. Levinson
Corrective action and preventive action (CAPA) is probably the most important process in any quality management system because so much else depends on it. This includes not only its traditional role as a response to defects, nonconformances, customer complaints, and audit findings, but also outputs…