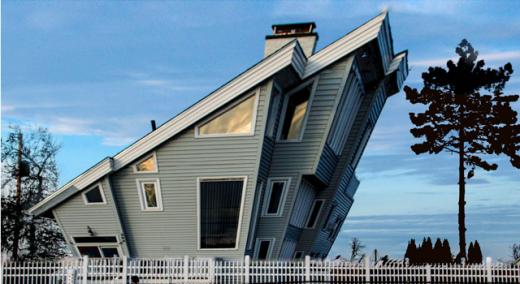
"New Age House" Credit: Russ Seidel
The computation for skewness does not fully describe everything that happens as a distribution becomes more skewed. Here we shall use some examples to visualize just what skewness does—and does not—involve.
ADVERTISEMENT |
The mean for a probability model describes the balance point. The standard deviation describes the dispersion about the mean. Yet a simple description of skewness is elusive. Depending on which book you read, skewness may be described as having something to do with the relative size of the two tails, or with the weight of the heavier tail of a probability model.
…
Comments
Clarity!
Don,
Of the many articles you have written over the years on this subject, this was probably the clearest explanation yet. To those who insisted on disregarding the empirical evidence shown in your work (e.g., Normality and the Process Behavior Chart), this will hopefully provide a clear and technical enough explanation that should be more problematic to refute.
You should consider a new edition of Normality to include some of this recent work.
Congratulations!
Best regards,
Rip
Process Behavior Charts are easy
What will be upsetting for all those folk who did courses in the Six Sigma Scam and were told to buy expensive statistical software to account for skewness is that you have wasted your time and money.
Process Behavior Charts are easy for all employees. You can draw them manually.
Add new comment