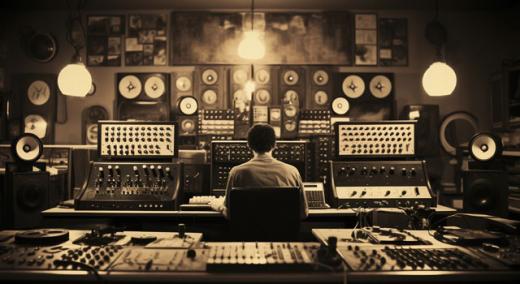
In last month’s column, we looked at how process-hyphen-control algorithms work with a process that is subject to occasional upsets. This column will consider how they work with a well-behaved process.
ADVERTISEMENT |
Last month we saw that process adjustments can reduce variation when they are reacting to real changes in the process. To be clear, I know that process control technology is everywhere, and it allows us to do things we couldn’t otherwise do. To borrow from Page 326 of my Advanced Topics in SPC (SPC Press, 2004), among other things, process-control technology allows us to:
1. Operate processes safely
2. Maintain process characteristics near a set point
3. Improve process dynamics
4. Reduce the effect of disturbances that can’t be economically removed
5. Accomplish complex control strategies
…
Comments
Excellent article
I shared this on LinkedIn. It underscores the fact that we cannot adjust random variation out of a process and, if we try, we will make it worse.
Box and Luceno wrote a book that combines process controller principles with SPC and they showed that you can in fact adjust "variation" out of a process, but only it it's a time series in which the long-term variation exceeds the short term variation (the portion measured by moving ranges on a chart for individuals). If you know what the time series looks like, you can apply process control algorithms to suppress the long-term portion of the variation but nothing can be done about the short term variation.
Add new comment