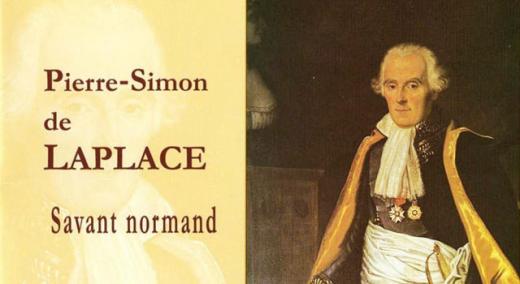
As the foundations of modern science were being laid, the need for a model for the uncertainty in a measurement became apparent. Here we look at the development of the theory of measurement error and discover its consequences.
ADVERTISEMENT |
The problem may be expressed as follows: Repeated measurements of one and the same item, if they are not rounded off too much, will frequently yield a whole range of observed values. If we let X denote one of these observed values, then it is logical to think of X as having two components. Let Y denote the actual, but unknown, value of the item measured, and let E denote the measurement error associated with that observation Then X = Y + E, and we want to use our repeated measurements to find an estimate for Y in spite of the uncertainties introduced by the error terms E. As it turns out, the best estimate to use will depend on the properties of the distribution of the error terms E.
…
Comments
Measurement Error
This article reminds me of an early undergraduate physics experiment conducted with my lab partner at Montana State University a long time ago. The purpose of the experiment was to measure the weight of an electron "n" times and report the result. I don't remember the value of "n" nor the variation of our individual measurements. What I do remember is that our report was downgraded because our average was too close to the accepted value. Measurement error was a constant during my 40 years in manufacturing.
Thanks Don and Quality Digest for this continuing series.
Electron mass measurement
Hi, Bill Pound (Pound? what a suggestive name for a metrologist!). When I was at college (Institute of Physics of University of Sao Paulo, Brazil) I had to determine the mass/charge relation of the electron too, the famous Milikan's Experiment. And the groups that got results very close to the actual value were always considered suspicious by the professor.
We had the accumulated results from the previous classes on the wall of the lab and the gaussian was pretty clear.
Add new comment