Getting Started with Process Validation Tools
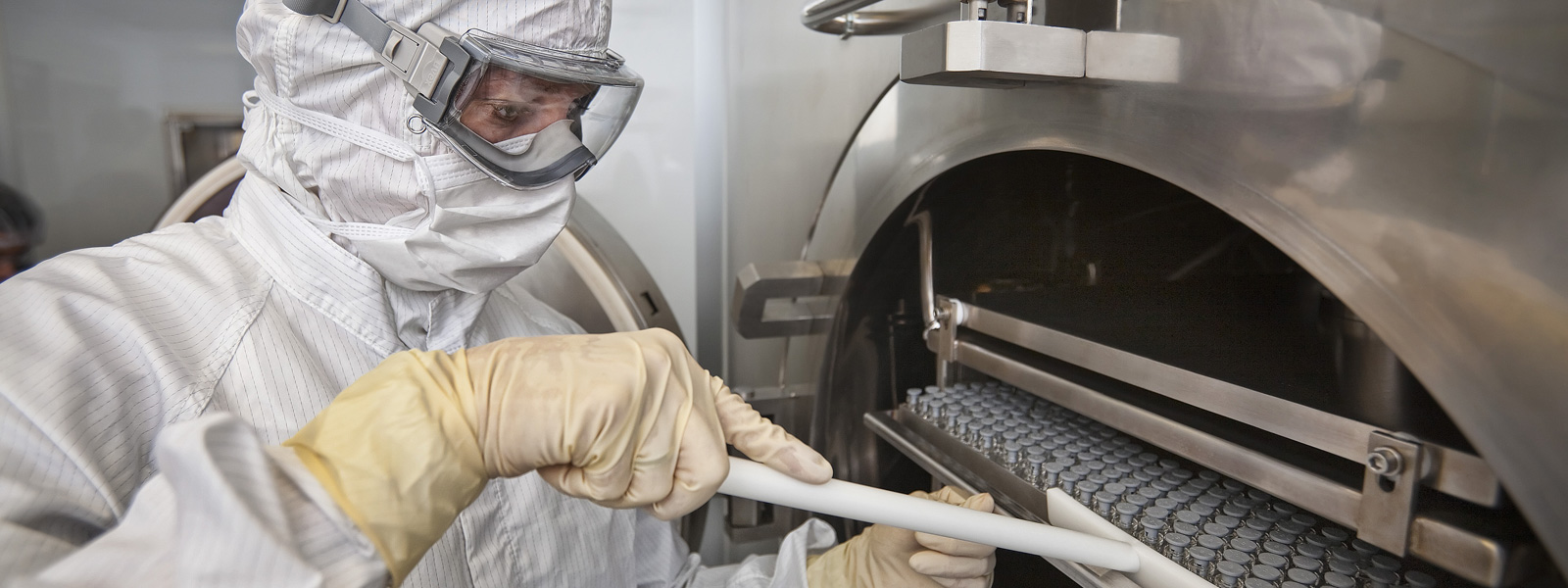
Process validation is vital to the success of companies that manufacture pharmaceutical drugs, vaccines, test kits, and a variety of other biological products for people and animals.
Process validation is vital to the success of companies that manufacture pharmaceutical drugs, vaccines, test kits, and a variety of other biological products for people and animals.
This is the second article in a three-part series to help readers distinguish good metrics from bad. In part one we discussed good metrics.
Perhaps the reader recognizes d2 as slang for “designated driver,” but quality professionals will recognize it as a control chart constant used to estimate short-term variation of a process.
Choosing the correct linear regression model can be difficult. Trying to model it with only a sample doesn’t make it any easier.
In this episode we look at a history of quality, how you serve your customer in the housing industry, and what makes a good review.
Metrics are an important part of an effective quality management system (QMS). They are necessary to understand, validate, and course-correct the QMS. They should be used to verify that it is achieving the goals and objectives defined by management.
In this episode we look at data, data, more data, and then... engineering the perfect human?
In my first article, the merits and cautions of AS9138 c=0 sampling plans were discussed and a simple formula was provided to determine the required sample size to d
In my previous article, I discussed the merits and cautions of the “acceptance number” equal zero (c=0) sam
Aerospace standard AS9138—“Quality management systems statistical product acceptance requirements” was issued this year (2018), a few years after its accompanying guidance materials in sectio
© 2025 Quality Digest. Copyright on content held by Quality Digest or by individual authors. Contact Quality Digest for reprint information.
“Quality Digest" is a trademark owned by Quality Circle Institute Inc.