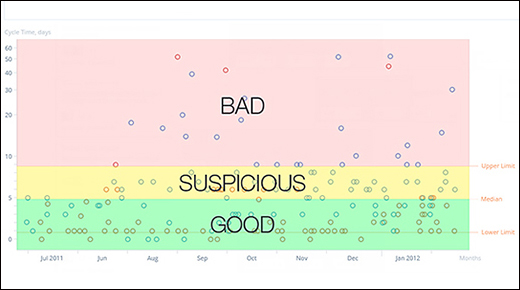
This is the second article in a three-part series to help readers distinguish good metrics from bad. In part one we discussed good metrics. Here, we will look at a bad metric and consider how to change it into a useful, good metric. A bad metric is one that fails in one or more of the attributes of a good metric and is often not usable for the purpose it was intended.
ADVERTISEMENT |
Attributes of a good metric
A good metric:
• Supports the goals and objectives of the quality system
• Contains data with sufficient detail to allow analysis of specific defects
• Contains data that have been carefully collected, and checked for accuracy and completeness
• Contains data that are combined in a way that clearly represents the process
• Uses a data-collection process that is clearly understood
• Demonstrates a clear relationship between the process and the data being used
• Has a metric-review interval that matches the response time for corrections
• Results in process improvement and overall cost savings
…
Add new comment