Content by Fred Schenkelberg
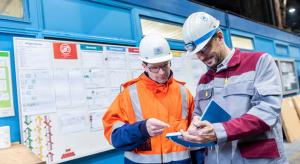
Wed, 04/28/2021 - 12:02
In 2019, Nicholas W. Eyrich, Robert E. Quinn, and David P. Fessell published an article in the Harvard Business Review titled, “How One Person Can Change the Conscience of an Organization.” In it, they discuss how corporate transformations, although…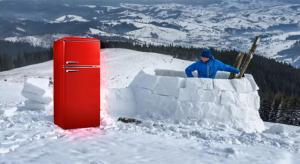
Mon, 02/22/2021 - 12:03
There is a type of error that occurs when conducting statistical testing: to work very hard to correctly answer the wrong question. This error occurs during the formation of the experiment.
Despite creating a perfect null and alternative hypothesis…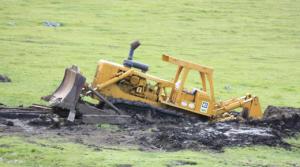
Wed, 07/18/2018 - 12:02
Reliability activities serve one purpose: to support better decision making. That is all they do. Reliability work may reveal design weaknesses, which we can decide to address. Reliability work may estimate the longevity of a device, allowing…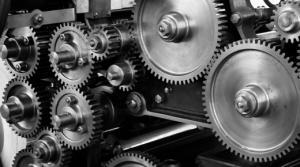
Tue, 05/08/2018 - 12:01
Mean time between failures (MTBF) is a symptom of a bigger problem. It’s possibly a lack of interest in reliability (which I doubt is the case). Or it’s a bit of fear of reliability.
Many shy away from the statistics involved. Some simply don’t…Tue, 02/06/2018 - 12:03
MTBF use and thinking is still rampant. It affects how our peers and colleagues approach solving problems, and there is a full range of problems that come from using the “mean time between failure” (MTBF) metric.
So, how do you spot the signs of…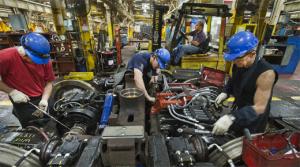
Mon, 11/13/2017 - 12:02
The term “Weibull” in some ways has become a synonym for reliability. Weibull analysis = life data (or reliability) analysis. The Weibull distribution has the capability to describe a changing failure rate, which is lacking when using just mean…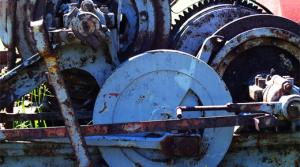
Tue, 08/01/2017 - 12:02
Our customers, suppliers, and peers seem to confuse reliability information with mean time between failure (MTBF). Why is that?
Is it a convenient shorthand? Maybe I’m the one confused, maybe those asking or expecting MTBF really want to use an…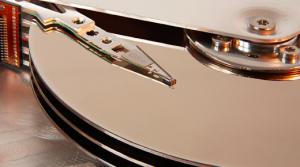
Tue, 04/18/2017 - 12:02
A conversation the other day involved how or why someone would use the mean of a set of data described by a Weibull distribution.
The Weibull distribution is great at describing a dataset that has a decreasing or increasing hazard rate over time.… Sample Size, Duration, and Mean Time Between FailuresUnderstanding reliability goals
Tue, 01/03/2017 - 14:51
If you have been a reliability engineer for a week or more, or worked with a reliability engineer for a day or more, someone has asked about testing planning. The questions often include, “How many samples?” and, “How long will the test take?” No… The Relationship Between Reliability Goals and Statistical ConfidenceFrom the desired state to a reflection of sample risk during measurement
Wed, 10/05/2016 - 00:00
We establish reliability goals and measure reliability performance. Goals and measures can be related; however, they’re not the same, and neither do they serve the same purpose.
Recently, I’ve seen a few statements that seem to confuse the role of…