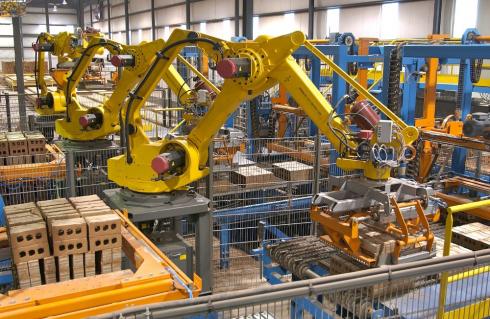
If you have been a reliability engineer for a week or more, or worked with a reliability engineer for a day or more, someone has asked about testing planning. The questions often include, “How many samples?” and, “How long will the test take?” No doubt you’ve heard the sample-size question.
ADVERTISEMENT |
What I continue to hear is the mistaken idea that adding another sample extends the effective time the testing represents in normal use. If I have a 1,000-hour test and add another unit, that doesn’t mean the results represent reliability for an additional 1,000 hours of use time.
The legacy of the exponential distribution
The problem stems from exponential distribution, where the chance of failure each hour for each unit is the same. There is no change to the hazard rate over time, therefore accumulating more individual hours provides additional information about how the system will behave throughout an hour of use.
This rarely if ever is true. Hazard rates change as different failure mechanisms evolve, as materials settle or wear, as damage accumulates, and as the environment changes. If you check, you will find assuming a content failure rate is invalid for your product or system.
But you know that.
…
Comments
Sample size in reliability studies
Fred,Good article. Just a comment: Just as in classical statistics where the precision of the estimate of a distribution parameter is a strong function of sample size, reliability parameter estimates behave the same way. That is, if you are trying to estimate any reliability parameter, be it the reliability at a specified time or the time associated with a specified reliability, the precision of the estimate is strongly affected by the NUMBER OF FAILURES, not by the number of units on test. In some special cases, such as in reliability demonstration tests with zero failures, we settle for a one-sided lower confidence limit on time or reliability (effectively with an infinite confidence interval width), but in general when a two-sided confidence interval or hypothesis test is required it's the number of failures that going to substantially determine the confidence interval half-width or the power of the hypothesis test. This conclusion applies to all reliability distributions, exponential or otherwise. So the first step of planning any reliablity study should be the determination of the required precision of the estimate so that the total number of failures required by the test can be determined. Then the number of units to be tested and their time on test follows from that.
Add new comment