Content by Davis Balestracci
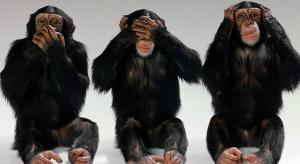
Tue, 07/14/2020 - 12:03
“With data from an epidemic there is no question of whether a change has occurred. Change is everywhere. The question is whether we are getting better or worse. So while the process behavior chart may be the Swiss army knife of statistical…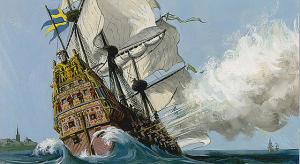
Mon, 06/08/2020 - 12:03
What is the Vasa? It was a Swedish warship built in 1628. It was supposed to be the grandest, largest, and most powerful warship of its time. King Gustavus Adolphus himself took a keen personal interest and insisted on an entire extra deck above the…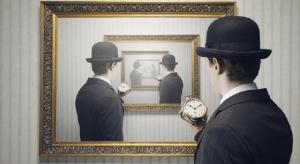
Thu, 01/16/2020 - 12:03
Editor’s note: The following browsable offering from Davis Balestracci represents a good chunk of his knowledge base. If you’re looking for improvement ideas, motivation, or a swift kick in the pants for yourself or your team, you’ll find them in…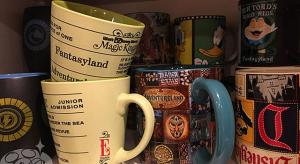
Thu, 12/12/2019 - 12:03
During the late 1970s, quality began to evolve from its historically Neanderthal, passive inspection approach to its current Cro-Magnon state, where its more proactive, project-based approach is bolted on to the operational status quo. Joseph Juran…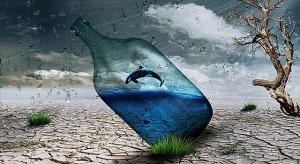
Wed, 10/30/2019 - 12:03
In 2006 I was at a presentation by a world leader in quality (WLQ) who has been singing W. Edwards Deming’s praises since the late 1980s and even does the famous red bead experiment as part of some of his plenaries.
He presented the following bar…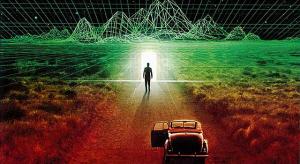
Tue, 09/03/2019 - 12:03
As statistical methods become more embedded in everyday organizational quality improvement efforts, I find that a key concept is often woefully misunderstood, if it is even taught at all. W. Edwards Deming distinguished between two types of…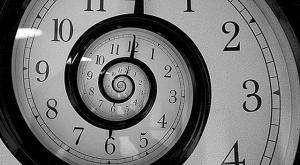
Mon, 06/17/2019 - 12:03
Recently, I’ve had a sad, increasing sense of déjà vu. Twitter has become even more vacuous, and LinkedIn has quickly devolved into a business version of Facebook. Literally right after I finished this draft, I read a newspaper headline: “Twitter…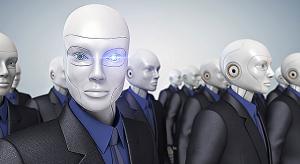
Mon, 03/25/2019 - 12:03
In most healthcare settings, workers attend weekly, monthly, or quarterly meetings where performances are reported, analyzed, and compared to goals in an effort to identify trends. Reports often consist of month-to-month comparisons with “thumbs up…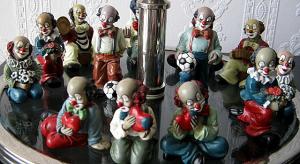
Thu, 02/21/2019 - 12:03
During recent visits to Twitter and LinkedIn, I’ve become increasingly shocked by the devolution of the posts to vacuous nonsense. I felt a Network moment of, “I’m mad as hell, and I’m not going to take this anymore!”
Is your organization getting…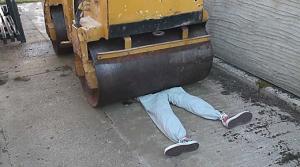
Mon, 01/14/2019 - 12:03
“People think that if you collect enormous amounts of data you are bound to get the right answer. You are not bound to get the right answer unless you are enormously smart.” —Bradley Efron
There has been an explosion in new technology for acquiring…