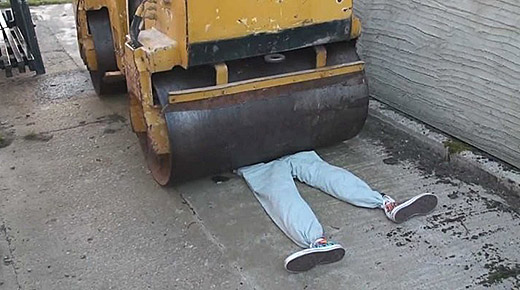
“People think that if you collect enormous amounts of data you are bound to get the right answer. You are not bound to get the right answer unless you are enormously smart.”
—Bradley Efron
There has been an explosion in new technology for acquiring, storing, and processing data. The “big data” movement (and its resulting subindustry, data mining) is becoming more prevalent and having major effects on how quality professionals and statisticians—and everyone else—do their jobs.
ADVERTISEMENT |
Big data is a collection of data sets that are too large and complex to be processed using traditional database and data-processing tools. Any change this big will require new thinking.
Rocco Perla, a colleague for whom I have the utmost respect, feels that even though there is now unprecedented attention and focus on analytics and data-driven decision making, it has also introduced a number of challenges.
…
Add new comment