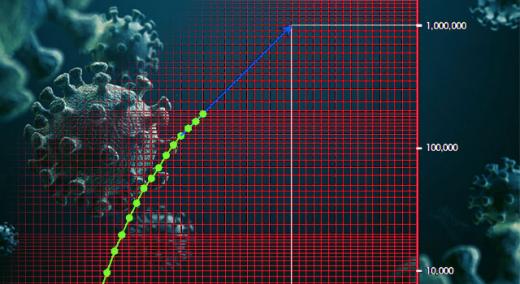
Based on the professional literature available, there are some inconvenient truths about Covid-19 that are not always considered in the chorus of confusion that exists today. Here we summarize what is known, what has already happened, and what is to be expected based on the analysis of the data and the epidemiological models.
ADVERTISEMENT |
Background
An analysis of the first 425 laboratory-identified cases of a novel coronavirus infected pneumonia (Covid-19) is presented by Qun Li, et.al.1. The first cases were identified at Wuhan hospitals as a "pneumonia of unknown etiology" when the patients met the following criteria: fever in excess of 100.4°F, radiographic evidence of pneumonia, low or normal white-cell count or low lymphocyte count, and no symptomatic improvement after antimicrobial treatment for 3 to 5 days according to standard clinical guidelines. On Jan. 7, 2020, the outbreak was confirmed as a new coronavirus infection2.
The analysis of these 425 initial cases revealed an average incubation time of 5.2 days, with a 95th percentile at 12.5 days. In its early stages, this outbreak had a doubling time of 7.4 days and an average time between successive infections of 7.5 days. For those who were hospitalized, the average time between the onset of illness and admission was 12.5 days. While 24 of the 45 cases identified by Jan. 1, 2020, were connected to the Huanan Seafood Wholesale Market, the closing of this market on that date did not stop the spread of the infection. Clearly this infection could be transmitted person-to-person among close contacts. On Feb. 11, 2020, The World Health Organization (WHO) named it Covid-19 and by March 11 had declared a pandemic2.
The Covid-19 Response Team at Imperial College London published on March 16 what one of our colleagues called "the best study so far available"3. This team used epidemiological models to look at how nonpharmaceutical interventions (NPIs) are likely to affect what happens in both the United Kingdom and the United States. We will discuss these NPIs and the team’s findings later. We mention this study now because it is the source for much of the following information.
The basic reproduction number for this infection appears to be in the neighborhood of 2.2 to 2.4 1,3. This is an estimate of how many new people in an uninfected population are likely to be infected by each person with Covid-19 on the average. For comparison, the seasonal flus have a basic reproduction number between 1.0 and 2.1. Analysis of data from China and from those returning on repatriation flights suggest that up to 40 percent to 50 percent of Covid-19 infections were not identified as such because of infections with no symptoms and persons with mild disease 3.
The infection fatality ratio (IFR) for Covid-19 is estimated to be about 1 percent, which is about 10 times that of typical seasonal flu. However, this is not uniform for all ages. Those in their 60s have an IFR of 2.2 percent. Those in their 70s have an IFR of 5.1 percent, and those in their 80s have an IFR of 9.3 percent3.
Tracking Covid-19
No data have any meaning apart from their context. When we are presented with numbers one value at a time we have trouble interpreting them. When we place those numbers in context they help us to understand where we have been and where we are going. When tracking the actual course of a pandemic this need for context is crucial. The situation is changing and the question is no longer "Has a change occurred?" but rather "How fast are things changing?" To this end we do not need anything more than a simple running record, but it helps to know what kind of running record to draw.
When working with exponential growth phenomena, the primary graph has always been the semi-log plot. The actual counts are plotted on a logarithmic scale while the dates are plotted on a linear scale. This plot preserves the nature and interpretability of the data since it plots the actual values, but it turns the exponential growth curves into straight lines. Since it is much easier to see when the slope of straight a line changes than it is to tell when a curved line changes shape, the semi-log plot is more easily understood. Moreover, it is easier to extend a straight line to make reasonable, data-based short-term predictions than it is to try to extend an exponential growth curve on a traditional graph4.
(For those who are not familiar with a semi-log graph, these graphs can be created in Excel using the scatterplot tool and choosing one axis to use a logarithmic scale. There is even a YouTube video on how to do this.)
Figure 1 lists the number of confirmed Covid-19 cases in the United States as reported by the European CDC5.
Figure 1: Number of confirmed Covid-19 cases in United States
These values show that in the United States the number of confirmed Covid-19 cases has increased a thousand-fold in 25 days (from 103 to 104,686 between March 3 and March 28). Since 2 raised to the tenth power is 1024, a thousand-fold increase represents 10 doublings. Ten doublings in 25 days gives an average doubling time of 2.5 days. The numbers in figure 1 double every two to three days throughout most of March. Figure 2 shows the data of figure 1 on a semi-log graph.
The straightness of the line shows a fairly stable exponential growth. However, the last few days show some slight change in the angle of the plot. Changes where the growth curve becomes more horizontal are known as "flattening the curve." Changes like this represent slower growth for the pandemic. Here the counts double between March 28 and April 2, so the current doubling time is estimated to be about five days.
Figure 2: Confirmed Covid-19 cases in the United States
What can be done?
While several pharmaceutical treatments are being tried, none have yet proven to be effective for Covid-196. Thus the only thing left is prevention which depends upon nonpharmaceutical interventions. There are five NPIs that were considered by the Imperial College task force when they ran their epidemiological models:3:
The first NPI model was case isolation in the home. Symptomatic cases stay at home for seven days. The second NPI model was voluntary home quarantine for 14 days for members of a household with a symptomatic case.
The third NPI model was social distancing of those over 70 years of age. This model assumed the reduction of workplace contacts by 50 percent and the reduction of other contacts outside the household by 75 percent.
The fourth NPI model was social distancing of the entire population. This model assumes this will reduce contacts outside the household, school, or workplace
The fifth NPI model was the closure of all schools and 75 percent of all universities.
These five NPI models were aimed at reducing contact rates within the population and thereby reducing the transmission of the virus. The microsimulation epidemiological models show that the effectiveness of any one intervention is likely to be limited, requiring multiple interventions to be combined to have a substantial impact on transmission.
The first three intervention models—case isolation, home quarantine, and social distancing of those at risk of severe disease—make up an optimal mitigation policy. Mitigation has the potential to slow the epidemic spread, reduce the peak healthcare demand by two-thirds, and cut the number of deaths in half3. However, since this will still overwhelm the intensive-care units and result in thousands of preventable deaths, the alternative of suppression has to be considered. Suppression has the aim of reducing the effective reproduction number (the average number of secondary cases each case generates) to below 1.03.
The epidemiological models for the United States show that suppression will require no less than social distancing of the entire population, home isolation of cases, and household quarantine of their family members. The major challenge of suppression is that this type of intensive intervention package will need to be maintained until a vaccine becomes available. If the interventions are relaxed, the models show that the transmission of Covid-19 is likely to quickly rebound3.
New Rochelle
New Rochelle is a suburban city of 80,000 in Westchester County which is just north of New York City. The numbers of confirmed Covid-19 cases reported by the Westchester County Health Department for New Rochelle are given in Figure 3.
Patient One for New Rochelle had been sick since February 27, but was only identified as being infected with Covid-19 on March 2. After his test came back positive several interventions were instituted, beginning with the closure of his synagogue, the quarantine of 100 families from that synagogue, and the tracing of Patient One’s contacts. On March 12, after 122 people had been found to be infected, an isolation zone with a one-mile radius from the synagogue was established by special order and all places where people could congregate were closed. The National Guard was called upon to deliver food to people sequestered at home and to clean gathering places within the zone. On March 13 a drive-thru testing center was set up near New Rochelle7. On March 25 the mayor announced plans to relax the isolation zone restrictions, but this was superseded by the governor’s stay-at-home order which was already in place.
Figure 3: Number of confirmed Covid-19 cases in New Rochelle
As may be seen in figure 4, the growth curve began to flatten out on March 7, after the initial surge. While the curve continued to climb, it was climbing much more slowly than previously.
Figure 4: Confirmed Covid-19 cases in New Rochelle
Between March 19 and March 30 the number of cases doubled from 172 to 346. Thus, these data show a doubling time for New Rochelle of 11 days. This doubling time is comparable with what is happening in countries that are doing a good job of mitigating the pandemic such as Japan, Taiwan, and Singapore5. (At this time, among those countries with more than 100 cases, only China, South Korea, and San Marino have suppressed the pandemic by getting the graph horizontal and reducing the effective reproduction number down to 1.0 or less5.)
A traditional way of looking at epidemiological data is to track the number of new cases each day. When we do this for New Rochelle we get the graph in figure 5. There we see three surges in the number of cases.
Figure 5: Number of new cases each day in New Rochelle
While the spread of Covid-19 was effectively suppressed for two short periods, each time it rebounded. Another aspect of the Covid-19 pandemic is illustrated by this graph—declines in new cases of Covid-19 lag behind the interventions. Accordingly, these data cannot be used to establish cause-and-effect relationships.
In addition, deaths from Covid-19 lag even further behind. On March 27 there had been a cumulative total of 9 deaths from Covid-19 cases in all of Westchester County. Three days later this total had climbed to 19 deaths. The next day, March 31, there had been a total of 25 deaths from this outbreak. All of this suggests that while non-pharmaceutical interventions can be used to mitigate, or even suppress, the Covid-19 pandemic, these interventions have to be maintained until pharmaceutical interventions become available.
What lies ahead?
Returning to confirmed Covid-19 cases for the U.S. shown in Figure 2, the slight flattening of the most recent points is encouraging. The last few days show a doubling time of about 5 days, which is better than the earlier doubling time of 2.5 days. However, Figure 6 shows that this doubling time still puts us on track to hit one million confirmed cases by April 14 or 15.
Figure 6: Confirmed Covid-19 cases in the United States
Hopefully we will continue to flatten this curve, but we have a long way to go. Effective mitigation will require a more rigorous adherence to the nonpharmaceutical interventions than we have practiced in the past. If we do not flatten this curve, when should we expect to hit 10 million confirmed cases?
Covid-19 fatalities
As of April 3, the United States had 6053 Covid-19 fatalities5. Figure 7 shows the plot of these Covid-19 fatalities. The projection arrow is based on the last seven values.
Figure 7: Covid-19 fatalities in the United States
If this curve does not get flattened further, we could have as many as 100,000 deaths as soon as April 17. Since this does not consider the impact of finite medical resources, we cannot continue to simply wait for things to improve on their own.
Download this Excel spreadsheet, enter the daily numbers yourself, and have a similar graph automatically updated for you.
Also, this simple Excel spread sheet should help show the difference between a linear vs. semilog chart for showing exponential data.
References
1. "Early Transmission Dynamics in Wuhan, China, of Novel Coronavirus-Infected Pneumonia," Qun Li, et. al., New England Journal of Medicine, v.382, No. 13, March 26,2020.
2. Liu Xia, Na Risha, Bi Zhengiang. "Challenges in the prevention and control of new coronavirus pneumonia" [J/OL]. Chinese Journal of Epidemiology, 2020, 41 (2020-03-28) <http://rs.yilgle.com/yufabiao/1186569.htm.DOI:10.3760/cma.j.cn112338-20…. [Internet pre-publishing].
3. "Impact of nonpharmaceutical interventions (NPIs) to reduce Covid-19 mortality and healthcare demand," Imperial College, London task force
4. "Single Subject Studies in Prostate Cancer: How Graphing the Data Can Provide Insight and Guide Clinical Decisions," Al Pfadt and Don Wheeler,
Oncogen Journal 2(2) 10, March 14, 2019.
5. Website of the European Centre for Disease Prevention and Control
6. "A Trial of Lopinavir–Ritonavir in Adults Hospitalized with Severe Covid-19," B Cao, et. al., New England Journal of Medicine, March 20, 2020.
7. "A Cluster Site Sees Progress After Isolation" Sharon Otterman and Sarah Maslin Nir, The New York Times, Saturday, March 28, 2020, vol.CLXIX, no. 58,646.
Comments
Thank you so much!
Through all of the media fog the three of you have allowed us to see. Thank you for recasting the numbers so that we can understand the importance of the nonpharmaceutical interventions. I wish you &/or Quality Digest would continue to publish the daily progress by updating the graphs as you receive the new data. One question comes to mind: is any of the data flawed by time lags or batch reporting from medical staffs that are currently too busy or overworked to keep up with "the numbers" while working (heroically) to save lives? Again, thank you and stay well!
The “quality” of our data
This is a very reasonable question. My ( Al Pfadt)response reflects my opinion based on my experiences in helping gather the data we reported in our article. Drs Wheeler and Whyte may provide other responses.
Real time data is always subjected to real world constraints, some of which I believed we addressed in our article.
For example, we report on the number of " confirmed" cases. Different sources can be consulted to obtain these data. In the United States CDC issues daily reports based on data they gather. We used a different data source from Britain which we identify in the article. As long as you consistently rely on the same data source this isn't a problem. Likewise our New Rochelle data comes from NYState reports that may " lag behind" data gathered at the local level and relayed to NYState for verification of the correct zip code used to assign it to one of 50 + counties in NYState, and more specifically to determine if that address is in New Rochelle or come other nearby municipality.
you will notice that our Figure 4, which shows the cumulative cases of COVID 19 identified in New Rochelle has 2 gaps associated with Saturday, February 28th and Sunday, February 29th. The following day's count included cases confirmed during that 3 day period. Up to that point, data had been updated during the weekend. No explanation was offered, but it is reasonable to assume that the demands of entering data during the weekend became too much for available staff. However, those data were gathered and transmitted to NYState officials so that the count is still accurate.
it is more complicated in the case of the New Rochelle data particularly to determine the relationship between the "true" number of cases and the "actual" number. Dr Deming and before him Dr Shewhart were quite explicit in stating that there is no " true" measurement for any naturally occurring phenomenon. This is particularly true for COVID 19, where there has not been an attempt to ascertain prevalence by any formal testing strategy because enough tests are still not available to do so. Accordingly, it is not possible to determine to what extent the rising number of " confirmed cases" is due to the spread of the virus or the availability of more tests to confirm cases that had been present for some time, or most likely a combination of both. However, a confirmed case is a confirmed case, regardless of whether or not others may have been overlooked. Likewise, due to the existence of false positives, the number of “ confirmed cases” may exceed the “ actual number” of cases. To complicate matters even further, there is a problem of false negatives which might result in the “ actual” number of cases being under reported. As long as uses the same operational definition of what constitutes a “ confirmed case” and uses that procedure consistently, there will not be any systematic bias in the number reported. Sorry for such a long answer but the question raised an important topic others must consider when interpreting our data or reporting their own.
Spread of reliable data collection...
"Real time data/real world constraints" clearly characterizes data collection in the USA at the State and County levels. We may yet see some data spikes that are not indicative of new cases, but rather show the challenges that local health departments are having in "catching up" with data reporting with limited staffs and over-stressed hospitals. The next sets of data that will become vital to restoration of "life-as-we-knew-it" will be around the global efforts to develop, test & deploy vaccines. Absent a vaccine that gains high public confidence, we will remain under the rules of the NPI's you mention. Again, many thanks for this work!
The future effects of a potential vaccine
Collectivrly, it feels like we are actors in the play " Waiting for Godot". We hope that the main character ( in this case either a vaccine or a cost/effective pharmaceutical intervention) shows up, but until then we are left without little else to do but bemoan our fate.
We know that social distancing works to not only curb the spread of the virus but to create a situation where 1 person infects fewer than 1 other person on the average. This creates a tipping point which leads to a state of affairs where people in a region aquire immunity faster than the become affected.
admittedly, this takes a prolonged effort that must be coordinated at the national level and requires that collectively we think about the welfare of other more than our own.
If anything positive comes out of this painfull experience it will be the reminder that " no person is indeed an island" and that indeed we are all in this together.
Therapies & vaccines...
Today, I watched with interest (on CNBC) as Bill Gates discussed the enormous challenge of researching, testing, getting approvals, manufacturing & distributing a vaccine at scale. Finding a vaccine that will be effective & safe for the people at highest risk iwill be quite an accomplishment. Even a 1‰ ineffectiveness and/or a 1% rate of manufacturing flaws could leave 700,000 -to 1.4 million people vulnerable. The time needed is largely due to layers of complexity associated with not only the science, but also the scale of manufacturing & distribution. It's all daunting & our current NPI's are truly the only way to buy that time. Fascinating statistics were the need not so desperate.
Article on Covid 19 tracking
Hi,
One of the factors that gets overlooked in my view is the effect of aggressiveness of testing on fresh infections growth. This was apparent in US where testing was absymally low in the early stages.
In India this is beginning to show now and will be a factor that will continue to change as such a large and spread out population coupled with resources to test keep improving for a long time.
What would be the metric?
Any comments and Has any study taking into account been done?
Regards,
Niraj Goyal
Reply to Niraj Goyal
Yes, the data are not perfect. In China they knew they had a pneumonia of unknown cause from those who were in the hospital, but testing of repatriated personnel showed that half o the cases were not identified initially because of low to no symptoms. So the confirmed cases are, in part, a response to the number of tests run. So this makes these data a lower bound on the actual number of infections. They are clearly not complete. But they are all that is available. So we analyze them to see what they can tell us. Is the rate of infection flattening out or getting steeper? If our non pharmeceutical interventions are effective the curve should flatten out.
Length of baseline period?
An interesting read. In parallell, I have used the numbers for the development here in Sweden for similar descriptions. One problem I have encountered is that numbers are obviously not necessarily stable. The numbers of cases or deceased simply fluctuate one day to another. There is a lag in reporting, so the most recent numbers cannot be trusted. But if you take most of the period except the last points, there is hope for a somewhat trustworthy series. However, you still have the problem of variation. How long period is needed to say that you have got a reasonably stable series? I would like to have a control chart. But how should such a chart be set up when you expect numbers to develop in an exponential pattern? Would taking the logarithm of absolute numbers be reasonable? Or is there any way to set up control charts with log scales?
Response for Robert Lundqvis
The transformations required to place limits around exponential growth curves unfortunately undermine the mathematical principle that underlies the filtration of noise. This makes limits on exponential growth curves so wide as to be usleless in practice. As explained in the article, the question is not whether a change has occurred, but rather one of estimating the rate of change that is happening. When plotting the daily values, this daily variation is more pronounced. When plotting the cumulative totals the plot stabilizes. Exponential growth shows up as a straight line on a semi-log plot, so when the points begin to all line up on one side or the other of the historic line we know that a change in the rate has occurred. The more points contained in a deviation from the previous straight line, the more credible is the interpretation that a change has occurred. In computing a new doubling rate it is more important to use the right data than to use an aribitrary amount of data.
Control Charts
Like another writer, I wondered if a control chart could not be used for the last 7 data points (on which the projections are made) to show if the rate of increase is stable and therefore predictable? If not is there a basis for the prediction other than "eyeballing"?
If nothing else a process behaviour chart would show that the increase is either stable or not stable?
thanks in advance,
Kim Boland
Reply for Kim Boland
On a semi-log plot any rate change must absolutely, positively, show up as a change in slope. If you can draw a stright line that passes through all of the last three or more points, then those points all represent essentially the same rate of change, and the extension of that line provides a context for interpreting future values. Points below the extension will represent a lower rate of change. Points above the extension will represent a higher rate of change. When interpreting a phenomenon that is growing exponentially, it is the rate of growth that is the question of interest.
Additionally, when working with cumulative totals we have the same smoothing mechanism in place that works to smooth out averages. So if we have only a few values in our total we may find tht the cumulative curve on a semi-log plot will wander around a bit. These changes in slope will tell us that the variation in the day-to-day values is still affecting the total, and that any attempt to draw a projection line is inherently uncertain. So the smoothness of the curve plotted will provide a graphic answer the question about the stability of the rates.
There are also issues with abberations in the data collection process. When groups of formerly uncounted cases are added to the database in a "one-time correction" there will be a discontinuity in the curve. However, except for the point of discontinuity, the slope before and after the break will tell the same story if the rate remains the same.
So, rather than trying to find a way to use our general purpose statistical tool of the process behavior chart, here we simply need to let the data speak for themselves. Does this require judgment? Yes, it does. But then any successful use of a process behavior chart also depends upon judgment in terms of rational sampling and rational subgrouping. When the changes are obvious to the naked eye, the running record is all that we need to see how things are changing over time.
Thanks for the question. Hope this helps.
Tracking COVID-19 log rates
Thank You Don!
You are my great statistical teacher and I can only confirm my own great findings in using logaritmic Y-scales with linear time scales for "practiacl trend tracing and prediction".
In my case it was the reduction of error rates in PPM as we learned and applied new technology for several design generations of base stations in the 80ies. Today's efforts being aimed at expanding use of 5G systems.
I did a small data based study of the COVID-19 here. I found that the arly average risk of death in Covid-19 was 100ppm of our 10 milj population, it is now up in 250 ppm. Also easy to see that it is 10 times more severe in death rate than seasonal flues. The death rate of app 1% of infected Covid-19 patients could be seen, but then the elderly home cases were not fully included. I, belonging to the male age interval of 70-79, have a natural statistical death rate of 30% in that time span. So an increase of 5%-points for my age group due to Covid´19 is of course worth being avoided by stying in house with my wife and business partner behind teams, zoom, whatsup, facetime and other new means of communication.
I see forward to your next article on COVID-19 trends in the US. Hopefully we will get our own curves for Sweden as the incremental data gets reliable and final.
Hakan Sodersved
Industrial Statistician
Stockholm
Sweden’s Covid 19 data
Hi Dr Sodersved
i just had an occasion to read your reply to our article published 6 months ago. I wonder if you have tracked your country's Covid 19 data using the methods you mentioned and if you could share these results with Don and me, as well as Quality Digest readers?Thanks for your kind words.
Add new comment