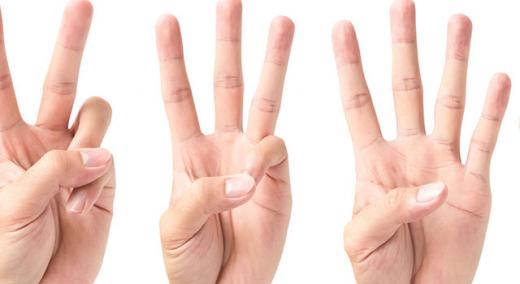
How many people drink pumpkin spice lattes in October, and how would you calculate this without learning specifically who is drinking them, and who is not?
ADVERTISEMENT |
Although they seem simple or trivial, counting queries are used extremely often. Counting queries such as histograms can express many useful business metrics. How many transactions took place last week? How did this compare to the previous week? Which market has produced the most sales? In fact, one paper showed that more than half of queries written at Uber in 2016 were counting queries.
Counting queries are often the basis for more complicated analyses, too. For example, the U.S. Census releases data that are constructed essentially by issuing many counting queries over sensitive raw data collected from residents. Each of these queries belongs in the class of counting queries we will discuss below and computes the number of people living in the United States with a particular set of properties (e.g., living in a certain geographic area, having a particular income, belonging to a particular demographic).
…
Add new comment