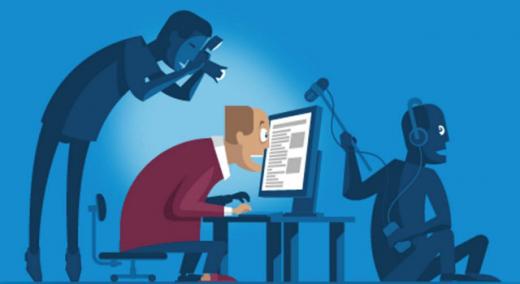
It’s not so simple to deploy a practical system that satisfies differential privacy. Our example in the last post was a simple Python program that adds Laplace noise to a function computed over the sensitive data. For this to work in practice, we’d need to collect all the sensitive data on one server to run our program.
ADVERTISEMENT |
What if that server gets hacked? Differential privacy provides no protection in this case—it only protects the output of our program.
When deploying differentially private systems, it’s important to consider the threat model—that is, what kind of adversaries we want the system to protect against. If the threat model includes adversaries who might compromise the server holding the sensitive data, then we need to modify the system to protect against this kind of attack.
…
Add new comment