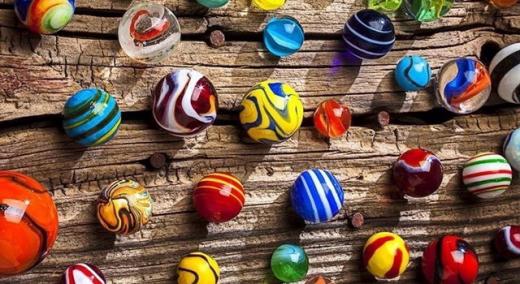
All articles in this series
Body
In part one we found the baseline portion of an XmR chart to be the best technique for identifying potential outliers among four tests with variable overall alpha levels. In this part we will look at tests which maintain a fixed overall alpha level regardless of how many values are being examined for outliers.
…
Want to continue?
Log in or create a FREE account.
By logging in you agree to receive communication from Quality Digest.
Privacy Policy.
Comments
x-mR chart as a homogenuity test
The idea of using x-mR chart as a tool to check homogenuity of data seems highly attractive to me, But, it contradicts the Shewhart's idea of "assignable cause" of variations. Shewhart wrote: "An assignable cause of variation ... is one that can be found by experiment without costing more than it is worth to find it. As thus defined, an assignable cause today might not be one tomorrow..." (SMVQC, p.30). By other words, according to Shewhart not all points outside the limits are outliers - some belong to the same system which produces the process data. So the question arises: how one can discriminate between points of assignable causes and outliers? Otherwise, if all points beyond the limits are outliers, assignable causes just disappear - this does not seem reasonable at all. Thank you in advance!
Add new comment