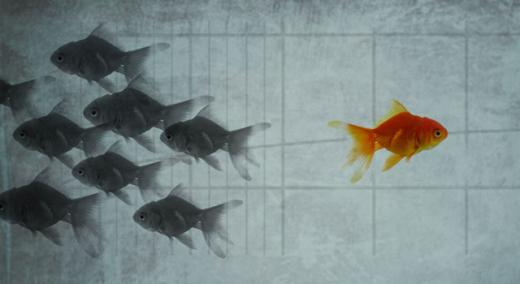
The first statistical test was a test for outliers. The problem of what to do about outliers has been around from the beginnings of data analysis. Part one will compare four tests for outliers. Next month part two will cover some additional tests for outliers.
ADVERTISEMENT |
Statisticians know how to analyze homogeneous data. After all, the initial assumption behind all statistical inference is that the data are represented by independent and identically distributed random variables. But all outliers are prima facie evidence of a lack of homogeneity. As such, outliers undermine statistical analyses. Hence statisticians often seek to sharpen up their analyses by deleting the outliers.
…
Add new comment