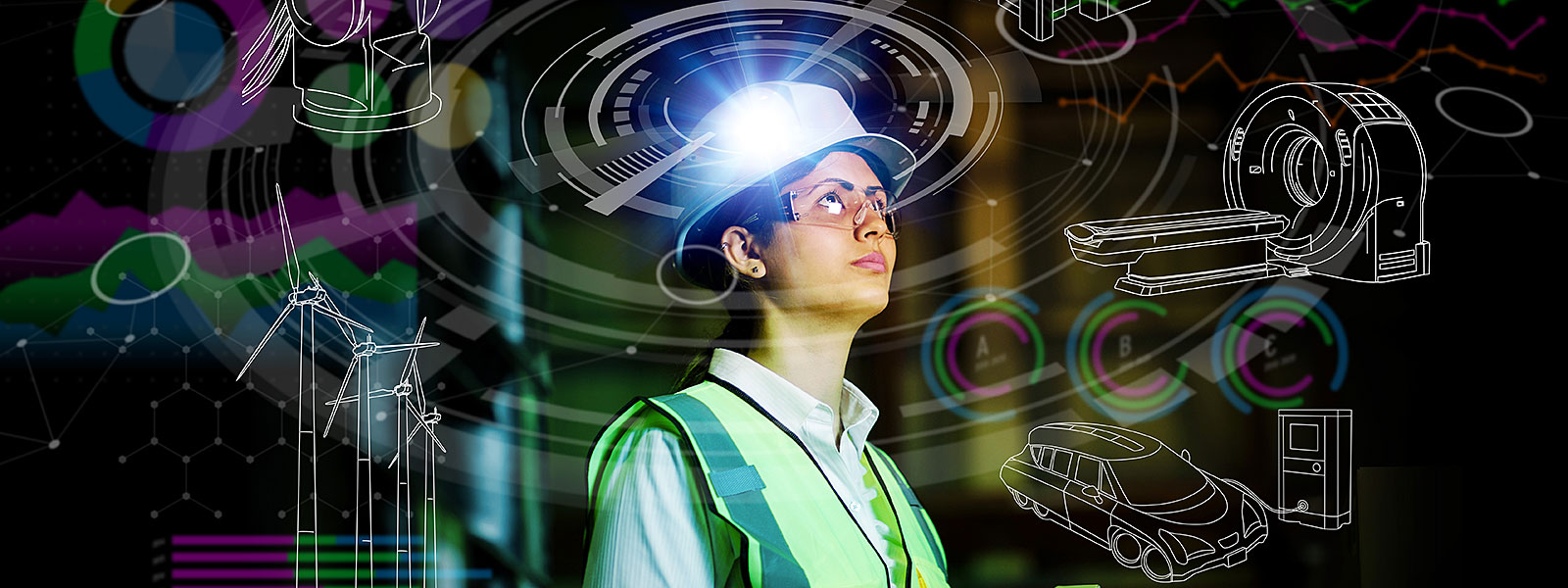
What is quality intelligence, exactly? It’s more than marketing spin. More, even, than the sum of its many control charts. It’s not collecting data simply to further go/no-go actions. And it doesn’t mean turning the cognitive wheel entirely over to artificial intelligence, either—far from it.
We might think of quality intelligence as a natural progression of quality control. It’s both granular, in that core quality tools underpin it, and forward-looking because quality data are used to improve not only products and processes but also operational performance. It’s very deliberate in that its goal is to wring the maximum value possible from reliable data.
To do this, quality intelligence employs four key tools: ensuring compliance, grading collected data, exploiting software, and implementing data strategically.
Ensuring compliance
People often assume that compliance applies solely to government or industry standards, but the term surfaces in many shop-floor conversations and processes. For instance, there is compliance to limits: Are data in specification? Are the appropriate statistical rules being met? There’s also compliance to procedures: Are people collecting data in the right way, and on time?
If compliance is the frame that supports effective processes and quality products, control charts are the nails that fix the frame in shape. To extend the metaphor, the more complicated a process or product, the bigger the frame/compliance, and the more nails/charts that are required. Therein lies a perennial quality quandary: Because every nail/chart must be shaped and tested to erect a reliable frame/compliance, there’s an inevitable human time limit to the endeavor. Too often what ends up happening is quality engineers spend their time budgets creating and checking charts to keep the critical compliance requirements in spec.
But what if those engineers had access to a screen where relevant SPC charts constantly rearranged themselves so that the problem ones showed up on the left-hand side? Wouldn’t that save time, and more important, brain power, to make operational decisions based on those charts? Does that sound like better use of engineers’ skills rather than riding herd on the charts themselves?
“This is why the current ‘old’ way of thinking, by looking at the control charts—or even worse, looking at the data—is flawed,” laments Eric Weisbrod, vice president of product management at InfinityQS, an SPC software and services provider. “There are simply too many charts and data values to look at, and the strategic value of the data can get lost.” That’s why Weisbrod, who began working at InfinityQS as an applications engineer, promotes quality intelligence as a logical and necessary response for modern industry. The company’s Enact software was created to address this issue so that organizations could steer toward a more comprehensive way of acting on their quality data.
“We saw organizations trying to do the right things and asking the right questions, but not being as effective as they could be in dealing with their increased visibility to data,” he says. “Using software to sift through the volume of data is critical because it’s too much to expect for an organization to do manually.”
As an example, he points to the chart in figure 1, from Enact, which shows expected yield vs. on-time checks for several different sites. This “bubble” chart summarizes data from multiple control charts across a company so that compliance to limits as well as time are available at a glance. It enables appropriate decisions to be made at an organizational level. An additional benefit of this visualization is that these summary results can be animated through time to see how yield and on-time collections change.
Figure 1: Bubble chart showing expected yield vs. on-time checks for several different sites (bubble size represents the amount of data for that site). Clicking on an individual bubble reveals more details.
Similarly, figure 2 shows a collection of notifications that are relevant to the current user, including a specification limit violation, a statistical violation, and both a due and missed timed-data collection. This type of chart is nothing new, Weisbrod says, but it can be invaluable when used in a specific and strategic way: “Set up your rules in the system, and let the system tell you when to take action,” he says. These notifications are the starting point for further investigation. Additional actions such as adding comments, selecting assignable causes, taking images, and viewing relevant control charts can all be accessed from these notifications. “This can work at all levels of an organization but tends to be a site-focused solution,” he says. The goal is to reveal meaningful insights in the data, a focus that gets to the heart of quality intelligence.
Figure 2: Notifications provide users with quick access to relevant issues.
Notifications are an excellent way to address specific issues, but how can an organization be more proactive with the quality data it collects? Which sites are performing the best? Where are there opportunities for easy wins? Where are the areas that need the most help? It’s for this reason—to prioritize resources and activities—that Enact includes a data-stream grading feature.
Grading collected data
Collecting metrics based on yield is the foundation of grading. These metrics can be used to analyze data streams to assess their expected and potential performance. Using the grading function in Enact, for instance, a manufacturer could tell which sites were operating reliably, and which had systemic issues. Armed with that knowledge, it would then be possible to examine a specific site more closely to identify which processes needed attention. Conversely, a manufacturer could use this technique to surface processes that were performing well and could be offered as benchmarks for other, similar processes across sites.
Grading is meant to serve as a culmination of diverse data streams for the sole purpose of acting on those data in an enlightened way. As such, its components are formatted in simple, color-coded chunks of information—A,B,C, and so forth, much like a report card. In this way, grading can provide both site-level overviews and more detailed analysis. Examples of a grading matrix and an “easy win” A3 distribution are shown in figures 3 and 4, respectively.
Figure 3: A grading matrix allows drilling into all data streams across an organization that exhibit different process potential and performance behaviors.
Figure 4: An “A3” grade is defined as being capable of achieving 100-percent yield (that’s the “A” part of the grade) but is performing at less than 90 percent of that potential (that’s the “3” part).
“People tend to think of data’s immediate use for process control, but the bigger wins are in data’s strategic insight,” says Weisbrod. “Being able to see multiple plants, pulling data from a wider range and over time, is a way to leverage greater value from all the quality information available.”
“This leads to organizations asking questions like, ‘What is the Cpk of my site?’” says Weisbrod “The spirit of this question is sound, but trying to determine this metric is fundamentally flawed.” Data stream grading was created so it could be rolled up; by making sense of all the data it collects, an organization can know where to effectively prioritize its resources (see figure 5). Employing yield-based metrics on critical characteristics is an effective way to roll up data across a site so sites can be meaningfully compared. Using simple A/B/C and 1/2/3 grades, emphasized with colors, allows users to directly drill in to the sites of interest.
Figure 5: A site‘s summary tiles for key characteristics allow a user to quickly identify areas of interest and prioritize resources to address issues and leverage opportunities.
Exploiting (and trusting) software
There’s still a limiting tendency in manufacturing to behave both cautiously and, at times, obstructively around SPC software. People want to know how their computer arrives at its solution, partly out of mistrust and partly out of curiosity, both traits hardwired into us. But does a person really need to see a control chart to benefit from its analysis? Most of us would say yes.
More so than ever, computers can crunch the numbers to analyze control charts or other rules better than we can. This is particularly useful considering the amount of data generated by the average production facility today. Given the complex operating axioms of modern manufacturing, operators and engineers need to delegate their chart-sitting so they can make better use of their time. They will need to trust that the results served up by their computers are accurate. There’s nothing mythical going on behind the scenes; the software is just taking over the time-consuming task of creating the charts and summarizing their data.
“Many operators are used to seeing control charts and interpreting them,” says Weisbrod. “Traditional SPC tools like control charts and histograms are still relevant, but how you get to them needs to change. With more data come more charts, and we have seen cases where users are either overwhelmed by them and just don’t look at them, or they don’t look at the right ones.”
This obviously has repercussions for performance. “More charts mean more processing effort and more time needed for a user to scan through the charts,” says Weisbrod. “Both of these slow down a user’s interaction, which is counterproductive for manufacturing.” Having a visual of the data is a “feel good,” but how many operators can truly understand, interpret, and respond correctly to every control chart in a complicated manufacturing process?
However, we can’t help trying to improve things that look “off” to us.
Say, for example, a trend rule, “six or more in a row increasing or decreasing” has been established. If you show most people a control chart with only five points ascending and ask, “Should the operator adjust the process?” Most of us would answer yes. Not so much because the process is in trouble, but because it looks like something might happen. Our human brains anticipate an issue, and our fingers itch to correct it.
“Next-generation SPC is still SPC; it’s just dividing the workload to make humans more efficient,” says Weisbord. “Statistical rules should be configured properly for the part, process, or feature being charted. Once these are set, they should be responded to only when violated. Let the computers do the more mundane tasks like looking for those violations, and let the humans do tasks they are better suited to, like problem solving.” Figure 6 shows a data-stream summary tile where each image represents a control chart analysis, and all data streams are sorted based on the violations in the data stream. The operator’s goal is to have an empty tile, meaning none of their processes have violations.
Figure 6: Stream summary tiles represent a control chart analysis. The data streams are sorted based on how many violations exist in that data stream.
Strategic use of quality data
Every organization understands the need for collecting data for the sake of compliance. Many see the tactical value in using these data to maintain the quality of their products and to control their processes. However, few organizations recognize the strategic value of those same data to improve quality and process performance across manufacturing operations. Or if they do, they often lack the tools to do so. SPC software like Enact allows them to take action, add value, and adhere to the core criteria of quality intelligence. These five criteria are fundamental:
• Data must be captured electronically; quality intelligence can’t be accomplished on paper.
• To be effective, data captured for an organization must all reside in a single repository.
• Data must be analyzed in a way that is clear to the user, visually engaging, and provides meaningful roll-ups and comparisons.
• Data from different processes, products, and manufacturing facilities must be treated consistently.
• Users of a quality intelligence system should focus on summaries of quality data and exception reporting.
Just like the other quality tools we’re all used to, no quality intelligence tool stands completely alone. It is when the right tools are combined that we are able to extract the most information. Figure 7 shows several dashboards using different tools to display data relevant to different users.
Figure 7: Dashboards can be configured to display relevant information to users at all levels of an organization.
We’ve looked at four different tools of quality intelligence and shown how they can be used. Operators, quality managers, quality engineers, and corporate quality professionals all have different, though related, needs. A company will get maximum value when all of these tools are used together across the organization. Effective software for quality intelligence should ensure that all of these stakeholders get what they need from the same underlying data, using the tools that are most relevant to them.
Add new comment