How to Master Quality: A Digital Transformation Approach
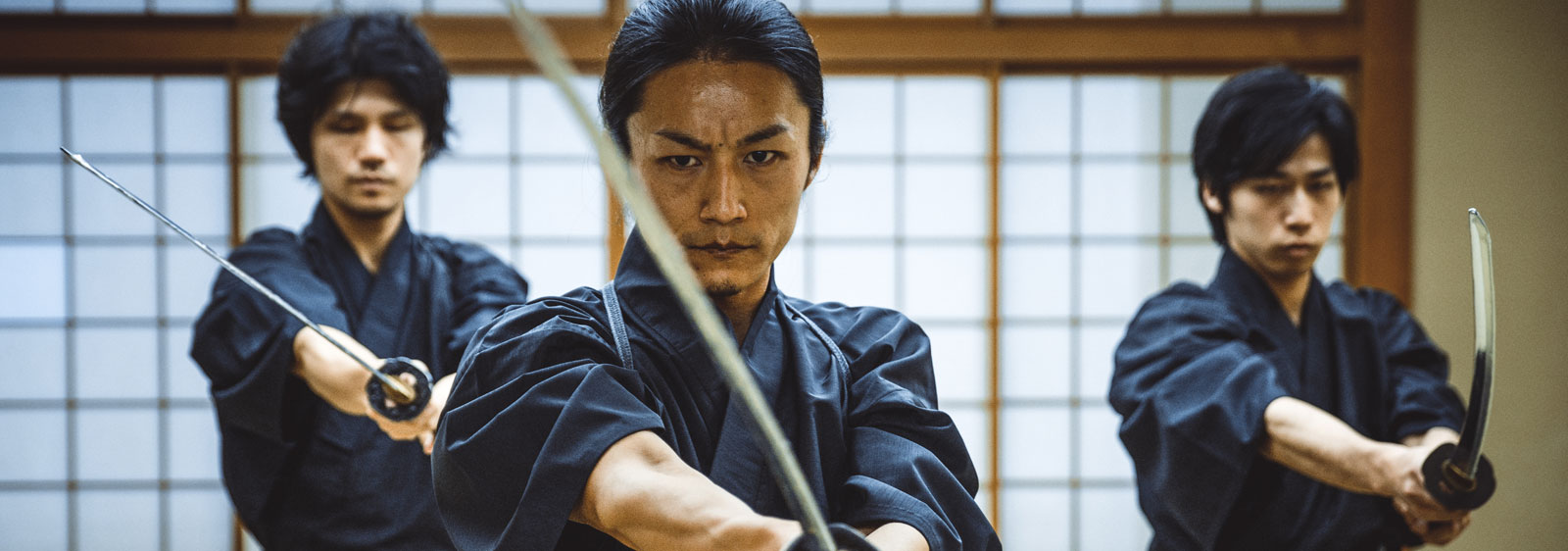
In previous articles of this series, we discussed how to master quality at the tactical and strategic levels.
In previous articles of this series, we discussed how to master quality at the tactical and strategic levels.
Before we get into a case study about how enterprisewide SPC software would work on both the shop floor and the C-suite, let’s talk about a long-held bias about “blue-collar” workers: That because they’ve traditionally been associated with manual labor, they should u
In order to best illustrate how enterprisewide SPC software can help address shop-floor problems and then funnel the captured data to the corporate level where strategic issues can be analyzed, here is a case study of a hypothetical manufacturing facility.
In recent months, we’ve learned that manufacturing during a global health crisis puts organizations under immense pressure to maintain operational efficiency while upholding product quality and employee safety.
Strategy and Tactics. Credit: "The Catch" by cas_ks
It’s been 40 years since “If Japan Can, Why Can’t We?”, W. Edwards Deming, and total quality management.
In the intro to this series we noted that, too often, quality tools and the data we glean from them are used only to solve immediate, mostly shop-floor problems.
If you're involved in business you know: Strategy matters. Your strategies guide you to reach your objectives. Behind every successful business are purposeful strategies.
This story was originally published by Knowable Magazine.
Imagine a science textbook without images. No charts, no graphs, no illustrations or diagrams with arrows and labels. The science would be a lot harder to understand.
This month I read Andy Kirk’s absorbing Data Visualisation 2, or to give it its proper title Data Visualis
Data are valuable assets, so much so that they are the world’s most valuable resource. That makes understanding the different types of data—and the role of a data scientist—more important than ever.
© 2025 Quality Digest. Copyright on content held by Quality Digest or by individual authors. Contact Quality Digest for reprint information.
“Quality Digest" is a trademark owned by Quality Circle Institute Inc.