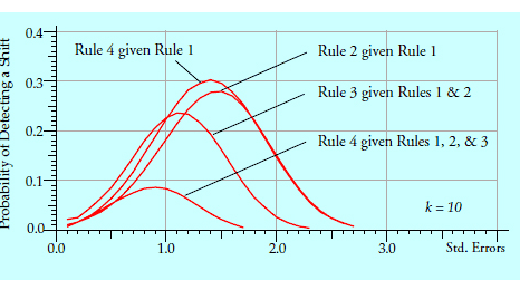
Body
How do extra detection rules work to increase the sensitivity of a process behavior chart? What types of signals do they detect? Which detection rules should be used, and when should they be used in practice? For the answers read on.
ADVERTISEMENT |
…
Want to continue?
Log in or create a FREE account.
By logging in you agree to receive communication from Quality Digest.
Privacy Policy.
Add new comment