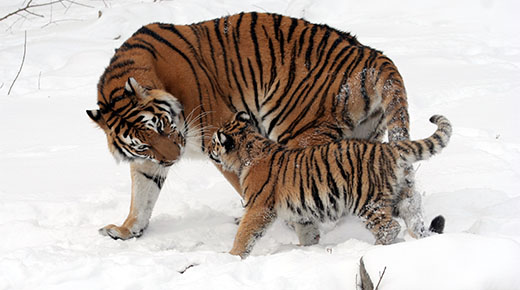
My last column mentioned how doctors and hospitals are currently being victimized with draconian reactions to rankings, either interpreted literally or filtered through the results of some type of statistical analysis. Besides the potential serious financial consequences of using rankings in the current craze of “pay for performance,” many hard-working people are stigmatized inappropriately through what has been called a “public blaming and shaming” strategy. Is it any wonder why many physicians are so angry these days?
ADVERTISEMENT |
A real example
Rankings with alleged helpful feedback to those allegedly needing it are also used as a cost-cutting measure to identify and motivate alleged poor performers. Many are analyzed and interpreted using an analysis that, based on courses people have taken, intuitively feels appropriate, but should actually be avoided at all costs.
…
Comments
Distributed folk
Reminds me of the fact that in the general population, 50% of people are below average intelligence. Those who argue are usually in this group. (Median is approximately equal to mean for IQ. Yes, and it's pretty normally distributed. ) I had an aquaintaince once, who was very excited that she scored 99% in an IQ test. Then we those who have IQ between 0 and 25 who are idiots; IQs between 26 and 50 are considered imbeciles; and those who have an IQ between 51 and 70 are considered morons. Does this mean it is better to be considered a moron than the village idiot?
Still, I would prefer my physician to be a genius rather than an idiot.
Ugh!
Based on what you cite as the proposal, the pharmacy administrator demonstrates a profound lack of understanding of variation. (I suspect there wasn't much curiosity to understand the system that generated the data, either.) In the span of about 15 seconds I went through disbelief, anger, and disgust as I recognize the very real impact decisions based on such superstitious nonsense have on people's lives.
You keep doing what you do, Davis. I am following in Dr. Wheeler's and your footsteps to debunk these methods, and help those open to listening with better analyses.
All the best, Shrikant Kalegaonkar (https://twitter.com/shrikale or https://shrikale.wordpress.com)
Whether or not you understand statistics.....
A statement in a previous Balestracci article sums up the situation"
"Whether or not you understand statistics, you are already using statistics!"
This a real problem and with sometimes dire and even deadly consequences.
As early as 1916, Walter A. Shewhart began to think about and do something about "data sanity."
Great to see Davis Balestracci expand on Shewhart's work. Is anybody listening? We darned well better!
"In the summer of 1916, Walter worked with the Western Electric Co. in New York (an integral part of the Bell Telephone system). He was examined by the medical officers of the firm who all thought he was suffering from tuberculosis. All tests were negative, but he had small fluctuations of temperature which seven medical men accepted as convincing proof of tuberculosis. Walter then started taking temperatures of other people, and found that his own father had similar fluctuations although he had never been ill except only once in his life. Walter discovered that most people have temperature fluctuations; so that his own case could not possibly be considered as abnormal, and he decided not to worry any further about T.B. This clearly was an authentic early piece of work in Quality Control."
Walter A. Shewhart and Statistical Quality Control in India Author(s): P. C. Mahalanobis Source: Sankhyā: The Indian Journal of Statistics (1933-1960), Vol. 9, No. 1 (Oct., 1948), pp. 51-60 Published by: Indian Statistical Institute
Add new comment