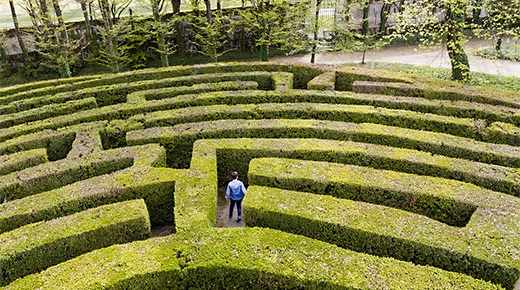
In The Music Man, the con man Prof. Harold Hill sells band instruments and uniforms and then tells the kids that they can play music if they will “just think about the notes and then play them.” In many ways this “think system” is similar to what you are asked to do with the define, measure, analyze, improve, control (DMAIC) approach to quality improvement.
During the define phase of DMAIC, you and your fellow band members are told to think about your process inputs and identify those inputs that have the greatest impact on the process outcomes. (As if R&D has not already done this.) To this end the tools offered in this “think system” are cause-and-effect diagrams, failure mode effect analysis, and designed experiments. To illustrate how this approach works, we will look at some of my clients’ experiences.
One client had a problem with the conformity of a high-volume product. Historically, 50 percent of their production had failed to meet the blueprint specification of less than 8 mils. Since 50-percent conforming would not allow them to ship enough product, the customer had agreed to a “deviated specification” of less than 12 mils. This allowed about 90 to 95 percent of the product to be shipped. The manufacturer and customer had operated with this deviated specification for several years, and they had found that the product worked satisfactorily in the field.
…
Add new comment