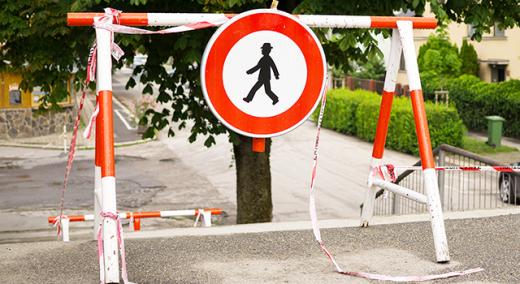
Measurement error is ubiquitous. As a result, over the past 250 years, different areas of science and engineering have come up with many different ways to deal with the problem of measurement error. One approach to the problem of measurement error was developed during the 1960s within General Motors. Over the years it was modified and revised until, in 1989, it was turned over to the Automotive Industry Action Group (AIAG). Since that time the AIAG Gauge R&R Study has been promoted throughout many different industries. Unfortunately, the original procedure contained some fundamental problems that have not been corrected over the years. This column will address these historic problems and suggest solutions.
ADVERTISEMENT |
The AIAG Gauge R&R Study
The AIAG Gauge R&R Study starts out with a sound strategy for collecting data. A simple fully crossed experiment is performed where two or more operators measure each of three to 10 parts two or three times apiece. To have an example to use we shall use the data shown in figure 1 where three operators measure each of five parts two times apiece.
…
Comments
Problems With the AIAG Gauge R&R Study
Thank you, Dr. Wheeler. Finally someone with the statistical credentials has said "the emperor has no clothes". Maybe now we can get AIAG to correct their error.
Also the RPN
Dr. Wheeler also pointed out the problem with the Risk Priority Number from FMEA, as it is the product of three ordinal numbers. The newest AIAG/VDA FMEA manual no longer uses it and has replaced it with an Action Priority. It is a major improvement on the previous method.
This article also has valuable takeaways. We need to know what is and is not of immediate practical value. I think the gage standard deviation (appraiser and equipment variation combined) is the most important because we can use it to calculate the chance of passing bad product and scrapping good product. The ratios, as Dr. Wheeler points out, are not as informative.
AIAG MSA Studies
Good article! I'd like to hear your take on a lesser known and practiced AIAG analysis, the (simple) Gage R. In the Gage R study, 10 measurements of a single characteristic (one part!) are taken by a single operator using a single measurement system. This would seem to eliminate part variation and (multiple) operator variation. The result being a distribution of the 10 measurements unsullied by operator (1, 2, or 3) differences, gage (micrometer?, calipers? CMM / CMM Program?) differences, or actual variation between parts (#1-#5). (What is included is "Within Part Variation", for example the difference in the thickness of the gasket at different locations. This variation can be minimized by requiring that the repeated measurement always be taken at a specific location.)
The Gage R analysis has been used extensively in auto-body panel production where it is important to reduce the spread of the 10 measurement distribution as part of the gage development long before quantities of production parts even exist. Note that these are not "easy" parts. They have few if any flat surfaces, are flexible far beyond their dimensional tolerances, and are likely to flex and/or move under measurement forces. Sophisticated holding fixtures (and a CMM) or gages (with integrated data collection) are essential for their measurement. Measurements are taken relative to coordinate systems structured around datums established from datum features designated on the parts.
The variation in the 10 measurements is sometimes severe. Depending on where and in what direction the measurements vary some conclusions for possible improvements can usually be made. Sometimes the part is moving on locator pins. Sometimes it is flexing (differently) each time it is measured. The insights gained by the analysis can even lead to tooling improvements that improve assembly fit and quality through the life of the program.
# of Distinct Categories
When you consider the range of values submitted to the Gage, I find that the number of distinct categories assists in explaining the cloud of uncertainty for a given value. Cloud = range / # of categories.
Dr. Wheeler, This article
Dr. Wheeler, This article was both insightful and comprehensive. I never miss one of your articles in Quality Digest. Thank you for continuing to educate and lead the quality profession.
Sincerely,
Jonathan Boyer
Gauge R&R
Suppliers will complain that their customers want the GR&R results using AIAG formulas.
Fortunately, it is easy to calculate the true results and provide them along side the AIAG ones.
Add new comment