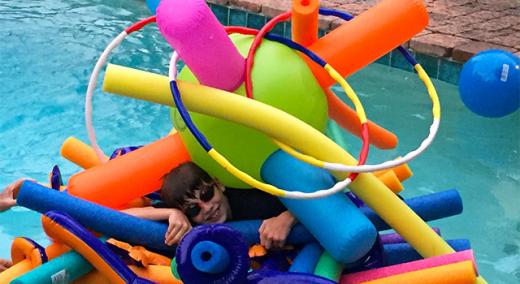
The oldest myth about process behavior charts is the myth that they require “normally distributed data.” If you have ever heard this idea, or if you have ever taught this to others, then you need to read this article.
ADVERTISEMENT |
While this myth dates back to 1935, and while Walter Shewhart exposed this idea as a myth in 1938, it continually reappears in various forms even today. For example, a white paper put out by a software company recently called the process behavior chart a “normality control chart.” And a blurb for a workshop advertised the Western Electric zone tests as “depending upon a normal distribution.”
As I consider how these myths are perpetuated I do not detect any malicious intent, just unconscious confusion. Nevertheless, those that continue to spread these myths fall into three groups. One group spreads these myths because of their special interests, another group spreads these myths because of their unexamined assumptions, and the third group spreads these myths because they were taught that the myths are true.
…
Comments
The Normality Myth
Very well written. I appreciate the class I took with you back in 1997.
Thank you for taking the time to write this.
Dr. Wheeler is a gem
I too took Dr. Wheeler's class in the 1990's. It forever changed my approach to numbers and statistics. The SPC Press training is pragmatic and easy to learn.
I just sent a new-hire to the class this week. Highly recommend over any other SPC training.
Bravo!
I'm often surprised by QIMacros users who think they have to check for normality and do a Box-Cox transformation to use a process behavior chart (i.e., control chart). I keep directing them to your wisdom on this topic. Thanks for another great article explaining it to the doubters.
Jay Arthur
QI Macros
I tried several years ago to get them (at QI Macros) to at least make it an option...they would not. As a result, I cannot recommend that package to clients.
Normality Myth
I agree that it is important to avoid the false positives, but I've found that it is equally, if not more important to capture the true positives. That is, capturing off-spec product when it IS off spec. So, when the distribution isn't normal, readings of -2 or even -1.5 sigma are indeed significant and indicative of a change while the positive sigma values indicate acceptable material. Missing a process change can be critical, depending, of course, on the process. As a result, I've found it useful to identify the distribution and set appropriate limits based on the probability. Then to convert those probabilities to the A and B values for operators. I'm sure Dr. Shewhart used the same A and B logic because he recognized the limited ability of QC professionals to make the conversions that are now so easily obtained with our PCs. Thank you for opening up this window on a poorly understood element of SPC.
Great comment Richard, I do
Great comment Richard, I do agree with what you are saying. Wheeler's points are tried and true and go all the way back to his seminal book with Chambers on "Understanding Statistical Process Control" written in the late 80's or early 90's. At the same time understanding the underlying distribution can be not only useful for identifying true positives on a process behavior chart, but also for understanding the physical nature of a process so you can further improve the process down the road.
Maybe I'm misunderstanding you here.
Control limits have nothing to do with whether the product is "off spec." Specifications are set by the customer. Just because your process has shifted in a positive direction, further within the specification, does not mean that this is acceptable. Quality means "on target with minimum variability," and the costs of your process being out of control are going to be passed on to your customers, who have to deal with incoming lots of product being different from previously-used product, regardless of whether the product satisfies specifications.
The point here is that you DON'T have to know the probability distribution for control charts to work. Unless you have a tonne of data about an extremely predictable process, you CAN'T know the distribution. Thus, you can't know the probabilities. OF COURSE we would like to avoid false negatives, just as we want to avoid false positives. But I'm sceptical that you have a statistical method for determining that a signal which looks exactly like noise is actually a signal, and that this method does not lead to more false positives than Shewhart's.
Normality Myth
Your point is well taken.
First, I apologize for my mistake in talking about specifications instead of control limits. I meant to say that there were occasions under Dr. Wheeler's examples where we would be making the error of assuming the process was in control when it actually wasn't. And this would be an issue only if the control limits were based on assuming a normal distribution when the underlying one wasn't. Some operations can be assumed to be normal . . . length, temperature, time, etc. Others can't, such as chemical purity or impurities in a batch.
One useful tool for processes with less than a tonne of data that I've found is the Weibull distribution which can help predict the expected distribution. Again, I should qualify myself and note that it isn't a universal solution. However, in the long run, I believe it is important to recognize that we often do make predictions that can be off by a country mile. Dr. Wheeler's calculations taken out to the nth decimal place need to be taken with a grain of salt, not because his math is wrong, but because of the difference between the real world and the mathematical world.
With this being said, I enjoyed the article and the coments from you and all the others. I learn from each of these articles . . . something that I couldn't do when I was younger and (thought) I was so much smarter! Thank you
Thanks!
Thank you for the thoughtful response. It is greatly appreciated.
Practical Limits to minimize false alarms in skewed data sets
If process data are truly independent and if one agrees to tolerate up to 2.5% of false positive signals, then one can ignore the underlying data distribution (symmetric or skewed) and be guided by 3 sigma limits as Dr. Wheeler suggests and advocates. However, there are situations where data distributions are inherently skewed and will inevitably entail a large and unacceptable number of false positive signals. Experience shows that these apparent outlying data can be considered as part of the common variation. An example would be microbial counts recorded in controlled rooms for production of sterile and non-sterile pharmaceuticals. In a regulated industry such the pharmaceutical one, every apparent out-of-control point must be investigated and documented under a strict quality system. When you generate hundreds of data, the "2.5%" can amount to considerable, annoying and costly futile investigations. Therefore, Richard Heller's approach to "identify the distribution and set appropriate limits based on the probability" is in my opinion understandable. To be practical, if the skewed data base is large enough and shows a recurrent and consistent pattern, one can try to either identify the exactly or approximately a data distribution or do empirical curve fitting and this will result in a much smaller amount of false positive signals. Shewhart used the terms "approximately" and “past experience” to state that a controlled phenomenon may be expected to behave in the future. So, if the data modeling reflects adequately or approximately the past behavior of a process, then I judge it reasonable to set what I call “practical limits” to such a skewed data set, limits that will minimize false positive signals. Furthermore, “past experience” can also reveal that certain skewed data sets, control limits based on 4 or 5 of 6 sigma limits can minimize false positive signals and in this case, these wider limits could also be viewed as another example of “practical limits”.
Normality Myth
Great article as per usual.
The distribution doesnt create the data!
The point is to try to make the best informed decisions on imperfect data, fitting models doesnt change this especially if the data does not display
homogeniety, yet another key and foundational use of the process behaviour chart which is so often ignored.
Thank you once again Don!
1935 and still going
Another great article. 84 years since Pearson thought he "fixed" what Shewhart overlooked. In 1931, on page 138 of Economic control of quality of manufactured product, Shewhart notes: "Pearson and his followers claim that these laws have been found to cover practically all cases coming to their attention." Was this a preemptive strike at Pearson's misunderstandings?
Any comments appreciated.
Thank you, Allen
Another great angle on this problem
Don always seems to find another angle; this one should, I hope, help convince some of those astute enough to understand. Personally, I was lucky enough to learn stats for analytic studies first via classes and practice in SPC (including some Wheeler seminars), and later learned the enumerative world as I got into DoE. The question of normality for SPC was never much of a question for me, and was settled for good once I read Normality and the Process Behavior Chart.
There is another aspect to the problem, though, and that is the fact that when you have time-ordered data, you cannot ignore the context of time. One of the things Shewhart did was to find a way to look for signals in time-ordered process data, recognizing that without stability, you cannot assume homogeneity. Without homogeneity, any distributional assumption is meaningless. It can very easily be shown (Don and Davis Balestracci have demonstrated this very well; I summarized Davis's argument in https://www.qualitydigest.com/inside/quality-insider-column/render-unto-enumerative-studies-073113.html back in 2013. In Davis's argument, he points out three different distributions that all test well for normality, but when you look at the time series, only one is stable.
So again, if you don't have homogeneity, you don't have any reason to assume any distribution. You can test for normality--it's just a calculation--but it would be meaningless if the time series is shifting or out of control. There is no distribution for data from an out-of-control time series. This aspect of the problem also makes testing for normality prior to examining the data in a chart a "cart-before-the-horse" exercise.
Normality necessity for Cpk calculation?
Hello all,
The Normality Myth article was great.
We learned that for SPC charts, normality is not necessary.
My question is that:
How much is it acceptable to use usual formula (normal distribution formula,3 sigma) for Cpk calculation when the distribution is not normal or is unknown?
thanks in advance.
How do you deal with excess false alarms?
In the article above, are we downplaying the significance of false alarms? For example, the article says the false alarm for exponential distribution is 1.83%, which is pretty high in my opinion. However, actual false alarm can be much higher, depending on how we calculate the contol limits. Here are my calculations:
I generated 10000 randon exponentially distributed data with scale =2 and threshold = 0.
The parameters of the random data set:
The derived parameters:
The false alarms expected using excel claculation =1-EXPON.DIST(UCL,1/2,TRUE)) is as follows
The false alarms observed from the random data set is as follows:
If the data was actually normally distributed, we should expect a false alarm rate of around 0.13%. In exponential distribution, the same approach will generate 22 TIMES more false alarms at approx 2-3%.While I agree that normality is not required as a test for most of the cases, but with inherently skewed data like exponential distributuion, be ready for false alarms upwards of 2-3% if we don't care about normality.If you are willing to live with 2-3% false alarm, follow Dr Wheeler's advice that XmR charts are robust enough to handle any distribution, else, consider a transformation like box-cox to actually identify the out of control points.If existing softwares like Minitab / JMP ? etc are too costly, you cal also explore ZOMETRIC Statistical module. It also offers Industry 4.0 ready automated / online / real-time control charts.
Ok... but
I've played around with modifying control charts with transformations when the data is far enough away from the normal distribution. My limited experience doing this tended to eliminate false alarms but also hid actual alarms. So there's a trade-off between false negatives and false positives. I've come to the conclusion that the simplest analysis that provides insight to the process is the best (to paraphrase Dr. Wheeler).
I'm curious - do you often run into processes that generate exponential distributions? If so, is there a particular type of process that we should look out for regarding this distribution? I could image machining operations might generate exponential distributions by nature of material removal. If this is the case, I would like to scrutinize that data a little more in the future.
Thank you.
Agree...
One of the most important principles that Shewhart tried to convey was that you needed to know your data. I have run across a number of processes that tend to skew positively over time (almost any cycle time data will present this way). When I have a set like that, I usually don't pay attention to, say, single data points just outside the upper limits.
On the subject of Box-Cox transformations: I have tended to view them with a high degree of skepticism, for the very reason Bulls mentioned. People often apply a lambda designed to get rid of the "outlier" values and warp the distribution shape into a bell shape. Trying to approximate homogeneity by forcing the distribution to "look" not only homogeneous but also normal calls into question (to me, anyway) both any signals (if they can show up) and any lack of signals.
Don did put together a couple of articles to talk about consistently skewed data (https://www.qualitydigest.com/inside/six-sigma-column/process-behavior-…). If you have enough data to be able to state with confidence that you have the situation I mentioned above, you can use that article to estimate an upper control limit that will probably handle the skew and reduce the false signal count with a little more precision than my "don't pay attention" method.
Add new comment