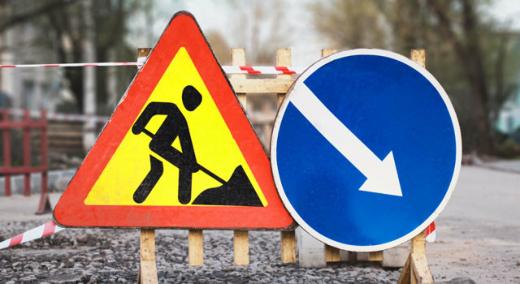
Ever since 1935 people have been trying to fine-tune Walter Shewhart’s simple but sophisticated process behavior chart. One of these embellishments is the use of two-sigma “warning” limits. This column will consider the theoretical and practical consequences of using two-sigma warning limits.
ADVERTISEMENT |
British statistician Egon Sharpe Pearson wanted to use warning limits set at plus or minus 1.96 sigma on either side of the central line. Others simply round the 1.96 off to 2.00. Either way, these warning limits are analogous to the 95-percent confidence intervals encountered in introductory courses in statistics. However, the use of such warning limits fails to consider the difference between the objective of a confidence interval and the purpose of using a process behavior chart.
A confidence interval is a one-time analysis that seeks to describe the properties of a specific lot or batch. It’s all about how many or how much is present. A confidence interval describes the uncertainty in an estimate of some static quantity.
…
Comments
But Wait, There's More...
Thank you for another excellent deep dive into why control chart rules work so effectively for sttistically stable processes.
I know you don't need to know this, but to me the other benefit of control charts over histograms is how special cause constitutes not only an alarm that something has changed, but quite often also offers information about what caused the change. The rules keep the decision objective, the visual display provides potential insight into the process.
Since you bring it up...
Since you mention histograms, it's probably worth noting that--in analytic studies, process studies--the histogram means nothing in the absence of statistical control.
Great reinforcement!
It costs, too...it's not as though you can use the two-sigma "warning" limits for free. Every false signal they pick up increases the chance that you will take action on a stable process. Those familiar with the Nelson Funnel experiment understand the dangers of that...tampering. It can increase the variation in a number of different ways, and will increase cost inevitably (you are paying someone to take action when no action is warranted or wanted).
I had a profound experience with this when I attended Dr. Wheeleer's "Advanced Topics in Statistical Process Control" seminar back in the mid-90s. He used a quincunx to demonstrate a stable process, and derived 1-, 2-, and 3-sigma limits from the data in the quincunx. Then he ran a lot of beads to show that the process was stable, and that his empirical rule held up. Then, he covered the top section so you couldn't see what was happening after the beads dropped through the funnel and drew specification limits on the board. Then he started dropping beads, and when a couple of beads started landing near a spec limit, some of the engineers in the room suggested that he shift the funnel away from the spec limit. So he did, then started dropping beads again. Every time a bead fell close to the upper or lower spec limit, he would shift the funnel (at the request of the students). After he'd done this for a couple of minutes, he pulled the paper off the front of the board and showed them that the distribution was significantly wider. Then he asked, "Do any of you use p-controllers in your processes? This is just a p-controller..."
This was well before the age of ubiquitous cell phones, and at the next class break, several of these engineers raced to the bank of cell phones out in the lobby, and called back to their factories to tell their people "Shut down the p-controllers! They're killing us!"
Is the process owner's intent
Is the process owner's intent to run a process that is in control or in compliance?
Control or compliance
Both. However, if it's not in control, you cannot predict that it will be in compliance.
2-Sigma Limits
I remember writing an article a few years ago about this same topic, though not nearly as sophisitcatedly as Dr. Wheeler. I ran into this problem many times in industry where someone in management felt the control limits were too wide. He just did not understand that the process determines the UCL and LCL, not the specifications or the desires of management. Long story short: These so-called "Action Limits" should be called "Tampering Limits"! "Tamper, tamper is the way. Off we go to the milky way."
Probability
The article states:
Can someone point me towards how these probabilities are calculated?
Three sigma limits are enough
Another great article by Wheeler. A little more detail is in his Advanced Topics in Statistical Process Control book. I thank him once again for repeatedly reminding us that 3 sigma limits are usually enough. In my organization it is easy to get a time series of data that shows a sign of trouble with just 3 sigma limits. The problem isn't a lack of signals, but a lack of action to identify and resolve the causes, preferably with prevention and not just reaction. So when people in my organization want (like I once did) more sensitive charts via runs rules (including the additional ones out there), CUSUM, EWMA, etc. I try to convince them otherwise. If you already have enough signals to work on, why do you think you need more? If a person is engineering a process I can see the desire for lots of signals, but one needs to remember the pain of chasing down the cause of something that was random (false alarm). Another statistician recommended DOE for understanding a process -- finding out what factors it is sensitive to -- so it can be made more predictable by acting on the lessons from such a DOE.
Add new comment