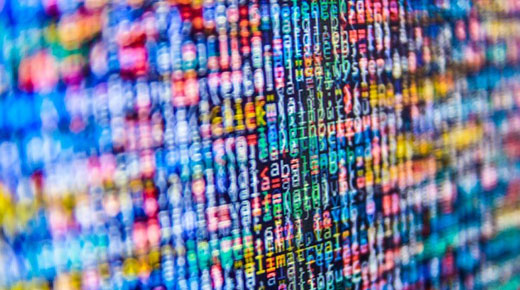
In Part One and Part Two of this series we discovered some caveats of data snooping. In Part Three we discovered how listening to the voice of the process differs from the model-based approach and how it also provides a way to understand when our models do and do not work. Here we conclude the series with a case history of how big data often works in practice.
ADVERTISEMENT |
Daniel Boorstin summarized the essence of distilling knowledge out of a database when he wrote: “Information is random and miscellaneous, but knowledge is orderly and cumulative.” As we seek to organize our miscellaneous data we have to be careful to make a distinction between signals and noise. The following is the story of one attempt to turn data into knowledge.
…
Comments
Data Snooping Part 4
Thank Dr. Wheeler for much needed paradigm shifts in the art of data snooping.
1. Medians are useful when exceptional variation exists.
2. Excessive variation can be predictable.
3. ANOM chart decision limits can be used on individual data eg.ANOX.
Dave Arnold
Add new comment