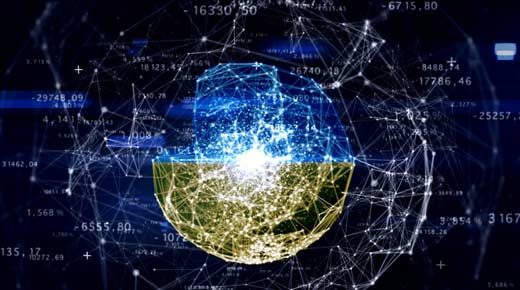
Data mining is the foundation for the current fad of “big data.” Today’s software makes it possible to look for all kinds of relationships among the variables contained in a database. But owning a pick and shovel will not do you much good if you do not know the difference between gold and iron pyrite.
ADVERTISEMENT |
When you start rummaging around in a collection of existing data (a database) to discover if you can use some variables to “predict” other variables you are data snooping (known today as data mining). With today’s software we can go snooping in very large databases in an effort to extract useful relationships. However, in the interest of clarity we will use a small data set and do our snooping using nothing more than bivariate linear regression. The issues illustrated here are the same regardless of the size of the data set and regardless of the techniques used to “model the data.”
Data snooping
The data set consists of five weekly production variables from a chemical plant. Figure 1 shows the data for a baseline of eight weeks of production. We will treat Y as our response variable, and see how well the other four variables do in predicting the value for Y.
…
Add new comment