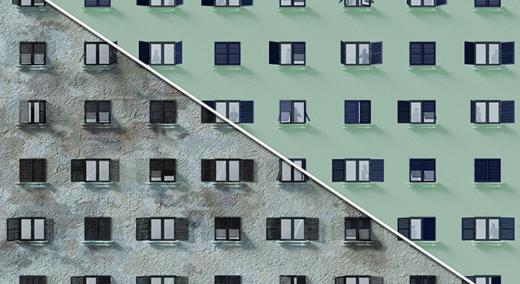
Last month we found that capability and performance indexes have no inherent preference for one probability model over another. However, whenever we seek to convert these indexes into fractions of nonconforming product, we have to make use of some probability model. Here, we’ll look at the role played by probability models when making these conversions.
ADVERTISEMENT |
Many people have been taught that the first step in the statistical inquisition of their data is to fit some probability model to the histogram. Here we’ll begin with the 250 values shown in figure 1. These values have an average of 7.4 and a standard deviation statistic of 5.2.
Figure 1: 250 observations from one process
…
Comments
Excellent clarifying examples
Digging the new photo, Dr. Wheeler.
And I absolutely love this content.
Such a detailed exploration that is subsequently summed up very nicely: "The numerically naive think that two numbers that are not the same are different. But statistics teaches us that two numbers that are different may actually be the same."
Add new comment