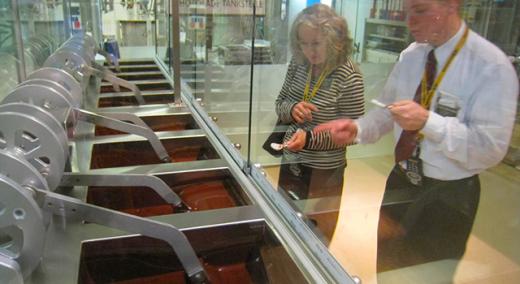
Recently I have had several questions about which bias correction factors to use when working with industrial data. Some books use one formula, other books use another, and the software may use a third formula. Which one is right? This article will help you find an answer.
ADVERTISEMENT |
Before we can meaningfully discuss different bias correction factors we need to understand what they do. To this end we must make a distinction between parameters for a probability model and statistics computed from the data. So we shall go back to the origin of our data and move forward.
A statistic is simply a function of the data. Data plus arithmetic equals a statistic. Since arithmetic cannot create meaning, it is the context for the data that gives specific meaning to any statistic. Thus, we will have to begin with the progression from a physical process to a probability model, and then we can look at how the notion of a probability model frames the way we use our statistics.
Assume that we have a process that is producing some product, and assume that periodic checks are made upon some product characteristic. These checks will result in a sequence of values that could be written as:
…
Comments
Important points!
We often say, "If there is one thing that everyone should understand..."
I remember Don stating in an Advanced Topics seminar that--shortly before he died--David Chambers told him something to the effect that if he (Chambers) had had it to do over again, he would do the ball socket/rational subgrouping exercise in every seminar they did. He thought it was that important.
The first few paragraphs of this article are something every Stats 101 student should be required to understand; they sort of describe some of the basic characteristics of what Deming called analytic studies. In most Stats 101-level courses, students are introduced to descriptive statistics, probability theory, and then distribution theory, but it's all in the context of enumerative studies, where we take samples from a (in principle) static population and extrapolate from those sample statistics to describe the population.
These concepts become the basis of everything else in college stats - a result of statistics being corralled under the school of mathematics at the beginning of the last century instead of the school of physical sciences where it belonged (I can't take credit for that; George Box wrote a paper about it, but I certainly agree with the sentiment). Tests of hypotheses and confidence intervals and most of the other concepts you learn unless you get lucky and take some industrial engineering classes taught by someone who undersstands analytic studies - all of these are based on that idea that there is a population and that we can describe some actual parameters of that population (at least for the time period of the sample).
Shewhart had another problem - the one most of us have in this game - the problem of process data, where time is an important context. Although Deming named it, Shewhart developed the idea of the analytic study, which was necessary because he was (as Don describes so well above) taking data from a dynamic stream, not a static population. In analytic studies we don't worry about populations, because they don't exist as a practical matter - you could argue that if a process is stable then there is a population of data that is developed while the process remains stable, but who cares? That would be semantic angels on the head of a pin (in my humble opinion). We are not trying to use a sample to represent and extrapolate from that sample to a population. We are trying to use subgroups to represent the past and present and extrapolate to the future.
If more authors who try to write about SPC understood this distinction, we would not have so many textbooks talking about "sample size" in control charts when they mean "subgroup size." We wouldn't have people writing that you have to test for normality (or for any other shape) before you establish that a process is in control and internally homogeneous (because only then can we assume that we have any distributional model at all to work with).
Thank you, Don, for reminding us again of this fundamental principle.
Add new comment