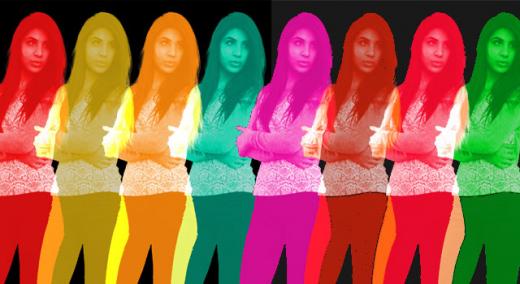
Adapted from "Repeat" by Morgan Wylie.
A simple approach for quantifying measurement error that has been around for over 200 years has recently been packaged as a “Type 1 repeatability study.” This column considers various questions surrounding this technique.
ADVERTISEMENT |
A Type 1 repeatability study starts with a “standard” item. This standard may be a known standard with an accepted value determined by some master measurement method, or it may be an item designated for use with the study (an “unknown standard”). The standard is measured repeatedly, within a short period of time, by a single operator using a single instrument. Finally, these repeated measurements are used to compute the average and standard deviation statistics, and these are used to characterize the measurement process. This technique can be traced as far back as Friederich Wilhelm Bessel and his Fundamenta Astronomiae, published in 1818.
The questions below pertain to how to use and interpret the results from a Type 1 repeatability study.
Question 1
Do we need to be concerned about the predictability of the measurement process?
…
Comments
Good Stuff
I look forward to Dr. Wheeler's articles every month.
I think we take for granted the wisdom he imparts for us in these pages. Kinda reminds me of a line from a Rush song, "bearing a gift beyond price, almost free".
Thank you, QD, and thank you, Dr. Wheeler.
Test Method 65?
What is Test Method 65?
Reply
Test Method 65 was the name of an assay at a chemical company lab.
It was short for Corporate Test Method, which I suspect means that it was developed in house.
Ditto
Well said and I agree completely. Most of my understanding of statistics and application to real-world problems has come from Dr. Wheeler's seminars and writings. What I learned from him has allowed me to make significant and lasting process improvements throughout my career. Dr. Wheeler is a gem.
Richard Lyday
Great paper, as always. I find it staggering that millions of people swallow the blatant Six Sigma trash rather than taking the time to read and understand Dr Wheeler's Quality classics. How could anyone in their right mind believe a process mean shifting +/-1.5 sigma was a good thing? Quality is in the Dark Ages. Dr Wheeler has the road to recovery.
I was curious about the Richard Lyday example. I see that a book was co-authored with him in 1984. I assume the vision systems mentioned were a pre-1984 variant? Today, vision systems are even being implemented by thousands of teenagers in the FIRST robotics competitions.
Type 1 Repeatability Studies
Another absolute gem from the master!
The fallacy of the typical approach to having confidence in the measurement process is laid bare here in an even more explicit and graphical way than previously.
Thank you Don!
Excellent article
I shared this to LinkedIn because many people don't really appreciate the importance of measurement system analysis. The phrase "rubber ruler" with regard to the stability of the measurement system is highly instructive.
More Excellence worth sharing
Good idea sharing this bit of wisdom. My "go-to" articles from Dr. Wheeler include:
"Two Plus Two is Only Equal to Four on the Average" This is a great article that I share with new hire engineers that recently graduated. It drives home the concept that numbers and calculations from those numbers are not always exact in the real world.
"Three Question for Success" Invaluable advice on continuous improvement efforts.
"A Better Way to Do R&R Studies" Debunks some of the concepts that are widely misused in industry and provides a meaningful way to evaluate a measurement system.
There are many more, but these 3 have had a huge impact on my approach to data gathering and analysis. They are readily available on the QD website and Dr. Wheeler's SPC Press website.
Add new comment