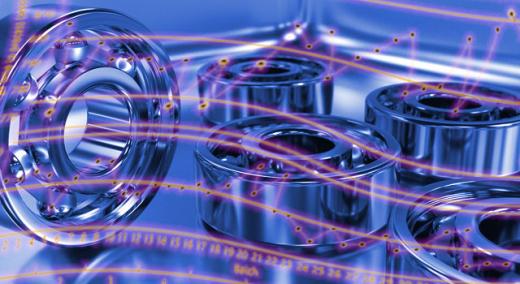
In everyday language, “in control” and “under control” are synonymous with “in specification.” Requirements have been met. Things are OK. No trouble.
ADVERTISEMENT |
“Out of control,” on the other hand, is synonymous with “out of specification.” Requirements have not been met. Things are not OK. Trouble.
Using this language, an obvious axiom would be: Take action when the process is out of control.
The everyday use of in and out of control is, however, unfortunate for control charts, the major tool of statistical process control (SPC). Why? Because in SPC these terms speak of processes as being stable or unstable. To characterize a process as stable or unstable, process limits, from process data, are needed. Specification limits are not needed.
Given the easy-to-understand basis for the action of meeting or not meeting requirements, coupled with the risk of confusion over the terms in control and out of control, why use control charts? If you are curious to see some of the benefits in doing so, read on. Two case studies are used.
Case one: Part thickness
During a regular review meeting in Plant 17, in- and out-of-specification data on the thickness of part 64 were reviewed.
…
Comments
Thanks, Scott.
Nice article. Working with a couple of colleagues responsible for incoming quality of subassemblies. This says what I've been trying to say much more cogently and interestingly.
We work for what's essentially been a huge job shop. Production articles have rarely been repeatedly produced, so we've been relying on engineering control (meeting spec) to assure we get what we want. Yes, yes, it would have been easy and helpful to use SPC and similar, but what we've done has mostly worked.
We've recently begun working with larger-volume manufacturers, working toward regular purchases involving significant numbers of the same parts, subassemblies, finished assemblies and even services. We've taken our usual approach of certifying an original design, then inspecting the bejeezus out of what comes to us and comparing that to specs. Many of the managers, engineers and others I've been working with are recognizing that we could get even more value if we make sure the manufacturing and operation processes going forward is the same as what got certified in the first place - that the process is predictable, in statistical control.
I'm from heavy industry. I know this will work. It's unfamiliar to my present employer and our suppliers, though. Time will tell whether it catches on in any meaningful way, but so far, the simplicity and head-smackingly visually obvious methods of SPC are piquing interest.
Keep writing. btw - really appreciate the relevant links to other articles.
"In control" does not mean you are meeting specifications
Good article except for your opening statement: "In everyday language, “in control” and “under control” are synonymous with 'in specification.' Requirements have been met."
Control charts tell us if the process is stable and predictable, not if requirements have been met. I've seen a lot of processes that are stable but not within specifications.
Once you have a stable process (determined by control charts), you can then use capability analysis to determine if the process is meeting specifications. Cp and Cpk can tell you if you're meeting specifications or not, as shown in this article.
Stable isn't always capable.
Two different meanings of in and out of control
Thanks for the comment. Fully agree that stable doesn't mean capable: In SPC, stable (in control) or unstable (out of control) tells us nothing about whether specs have been met or not.
The control chart meaning of in and out of control is different to the colloquial, everyday usage. I picked up on the potential obstacle of terminology some years back (e.g. https://www.spcpress.com/pdf/DJW129.pdf). My experience is that in control and out of control as terminology risks confusion, which can only reduce the expectation of successful control chart usage.
As an example, in training classes it typically works out that after breaks the sessions restart a few minutes late. I noticed it to be a pretty stable situation, repeating itself year after year and in different locations. I plan for it.
I learnt to make an analogy of re-start time as a “process”, where the goal is stated as an on-time start. This goal isn't met. When asked, a sizeable number of people tend to say this "process" is out of control (not in control). Yet, it is pretty stable, only far from capable. In Figure 10 this “process” would be in the Threshold State.
My emphasis at the start of the article is how in control and out of control are used in everyday, colloquial language. In everyday language, I believe "in control" and "under control" are synonymous with specs/targets having been met.
One set of terms and two meanings is a likely source of confusion. My point in the paper is that the colloquial use of in control / out of control has a meaning that is different to the “SPC meaning”.
Controlling understanding
In discussions with engineers, I've been careful to make a distinction between engineering and statistical control. Which, of course, uses the word "statistical", but one thing at a time.
I have a couple of canned charts showing how engineering control (being in spec, controlled by a feedback loop, etc.) is different from being predictable. We have lots of examples of weeding out half of a lot through inspection and high variation despite tight control of the wrong parameter to control to achieve quality to make my case. But it takes time and an engineer or a manager willing to stand with me in an elevator and talk about something besides the weather!
In these discussions, I've been forcing a connection between statistical control and predictability. Maybe it's time to rename statistical control to ... predictably controlled? Just predictable?
Well done Scott. Nice to see
Well done Scott.
Nice to see histograms without the all too common and meaningless normal distributions drawn over them
Your Fig 6 also shows how the WE Rule 4 (8 point below CL) is superfluous, as Dr Wheeler describes here: https://www.qualitydigest.com/inside/statistics-column/when-should-we-use-extra-detection-rules-100917.html "Rule one has been the key to process improvement for more than 90 years. Use it and learn about the dominant assignable causes affecting your process. In practice, rule one will usually generate all the signals that most people can realistically investigate."
Keep it simple. KISS. There's plenty of free software to draw control charts.
SPC
Dear Scott,
Thanks for such a detailed ,though simple way of presenting, today's most important topic of manufacturing processs-Why use control Charts!
I think, adjusting the average to the centre of the specification doesn't demand much effort in the process lines but manier times the reality is that we try to be too safer!
Am sure this article will help many of the factory guys to produce compliant product with stable process by operating at full potential.
Sukumaran
Understanding and reducing variation
A nice article by Scott.
Little's Law guarantees that if we reduce the variation in flow of materials through a process that either volume throughput will rise, or work-in-progress will fall, or some combination of both. This is a law.
Scott's article shows how using control charts helps us to understand and reduce variation.
Better quality, increased throughput, recuded cycle time ... we can have it all if we know how to reduce variation.
John McConnell
Hi John, I've had your books
Hi John,
I've had your books for decades.
I've added a variation slider in the Lean Simulation in Q-Skills3D, together with a graphical output. I've been rather surprised by the results. Variation has much less impact than I would have thought. The simulation variation slider varies the processing speeds randomly at either station. It would be interesting to see a mathematical analysis. Small changes in the mean at either station have an obvious effect. The sim also shows Little's Law graphically. It takes a long time to settle down.
Tony
Why use control charts
Hello Scott,
Very, very detailed article; shall need time to go through fully.
I only wish to express my surprise at two points of your article (as far as I have gone).
Sentence 3 i.e “Out of control,” on the other hand, is synonymous with “out of specification.”
AND
Whether figure 2 –plot of individual values of a parameter ‘should’ and ‘can’ be called a “CONTROL CHART”.
With due regards; I venture to disagree with both.
Kind regards
Virendra gupta
PS I shall read the article fully and try to give more comments.
Virendra, See my comments
Virendra, See my comments below on why I believe there a common language usage of out of control in relation to out of specification.
Control versus Predictable
Nice article Scott! You have articulated what is needed in production operations of every type.
I too ran up against the thought that "in control" meant within specifications. That is when I started using predictable and unpredictable to describe the behavior of a process. It has less emotional baggage than in control and out of control.
The problems in production are like fog or smog, and process behavior charts (control charts) can spotlight what needs attention and where we need to work. Moreover, without the framework of an approach such as control charts it is difficult to get the most out of any process. The problems of production are greater than those of research, where we spend a lot of time on developing techniques for learning. Moreover, the problems of production change with time, and this is why we need a time-oriented technique such as the control chart.
Thanks for this article.
Why Use Control Charts?
Thanks for writing the article, Scott.
It is unusual to see an article that is set in the real world and not the theoretical of SPC. Your approach is very practical. If only we can get more employers to see that SPC is meant to be used in realtime for defect prevention, rather than after the fact for process improvement, the world would be a better place. Fortunately, my employer firmly supports the former.
Why Use Control Charts?
Another great article, Scott. It is kind of sad there is still so much confusion about statistical control versus within specifications. Hopefully this article will be a helpful remedy. The Shewhart control charts put us at a crossroads and enable us to answer a very broad question: What problems will be best solved by workers (unstable) close to the process and what problems will be charged to management (stable)? Without this knowledge, management in general believes faults are on the factory floor and improvements are for someone else, not me. In The New Economics, Dr. Deming estimated 94% of problems belong to management and only 6% are special.
The big gains are in management awakening:
"Causes of nonrandom variation are called assignable causes or special causes. And those are usually chargeable to particular, local conditions that the workers can recognize and eliminate. And then you have left random variation that defines the system, and from then on only the management can improve it. That was Shewhart's great contribution." - W. Edwards Deming
Taken from:
THE EARLY INFLUENCE OF W. EDWARDS DEM1NG ON THE DEVELOPMENT OF STATISTICAL QUALITY CONTROL IN THE UNITED STATES AND IN JAPAN, Nancy R. Mann
link:
https://apps.dtic.mil/dtic/tr/fulltext/u2/p001590.pdf
Excellent material and detailed explanation of two cases
Hi Scott,
Thank you for our article. it is a great publication on two cases that brought the opportunity to discuss between the voice of the customer and voice of the process. Unfortunately, there are too many cases that there is no action until the trouble is already on our plate. As you mention, there is some missed opportunity to do not implement control charts and use them to translate this information to dollars.
European pharma is working on the similar area using multivariate approach. (https://www.edqm.eu/en/news/ph-eur-commission-consults-stakeholders-general-chapter-multivariate-statistical-process).
I look forward to your next article!
Marçal
Why Use Control Charts
I found this article especially useful. The Florida Department of Transportation is working with our crushed stone industry to implement statistical process charts. The industry uses a common commercial software with the capabilities, but SPC is not a familiar tool.
We are teaming to develop a system that is logical and works for both FDOT and Industry. A common case we have come across and are trying to assess is what happens when products are within spec, but the Natural Process Limits are outside the specifications. That is Cpk < 1. Scott's article makes it clear that this process is not stable or in control, but it is a difficult concept to grasp when we have to codify this situation in a regulatory document. We are considering allowing producers to take note of this situation and take corrective action. If so, FDOT would not take action against the producer. In our current system, we calculate the (difference between the average and the nearest spec), and divide the result by the standard deviation of the last runing 30 test results -absolute. This results in a Z score, which is required to be grater than 1.645 to ensure 95% compliance. In the situation described, the Z value would show that the data is out of complianc and the producer would be prohibited form shipping to FDOT projects. This method assumes all data are normally distributed. SPC does not and more closely matches our scenarios.
The article sheds light on a potentail solution to a real problem, and offers a system that might be practical and mutually benefical.
Add new comment