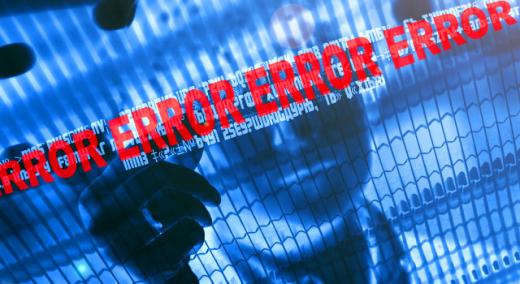
We are living in the digital age, and data have a universal presence that directly or indirectly affects our lives, even when we’re not aware of it. Hence, data quality is an important topic of discussion. Data quality isn’t only an aspect of data that determines their fitness for use, but is also a function or subdiscipline of data management.
ADVERTISEMENT |
Data quality can be defined as evaluating data’s fitness to use (that is, serve their purpose) in a given context. Sustaining high quality data is a challenge that most organizations face, and the data quality arena is surrounded by its own set of myths. This misleads people when it comes to making data quality management-related decisions. These myths can slow down, hinder, or put a stop to an organization’s data quality management efforts or the deployment of data quality projects or initiatives.1
…
Comments
Data Pedigree
I also recommend to read "Show Me the Pedigree", Quality Progress, January 2019.
Best regards
Three Comments
Good article. Here are my coments:
hi there
Add new comment