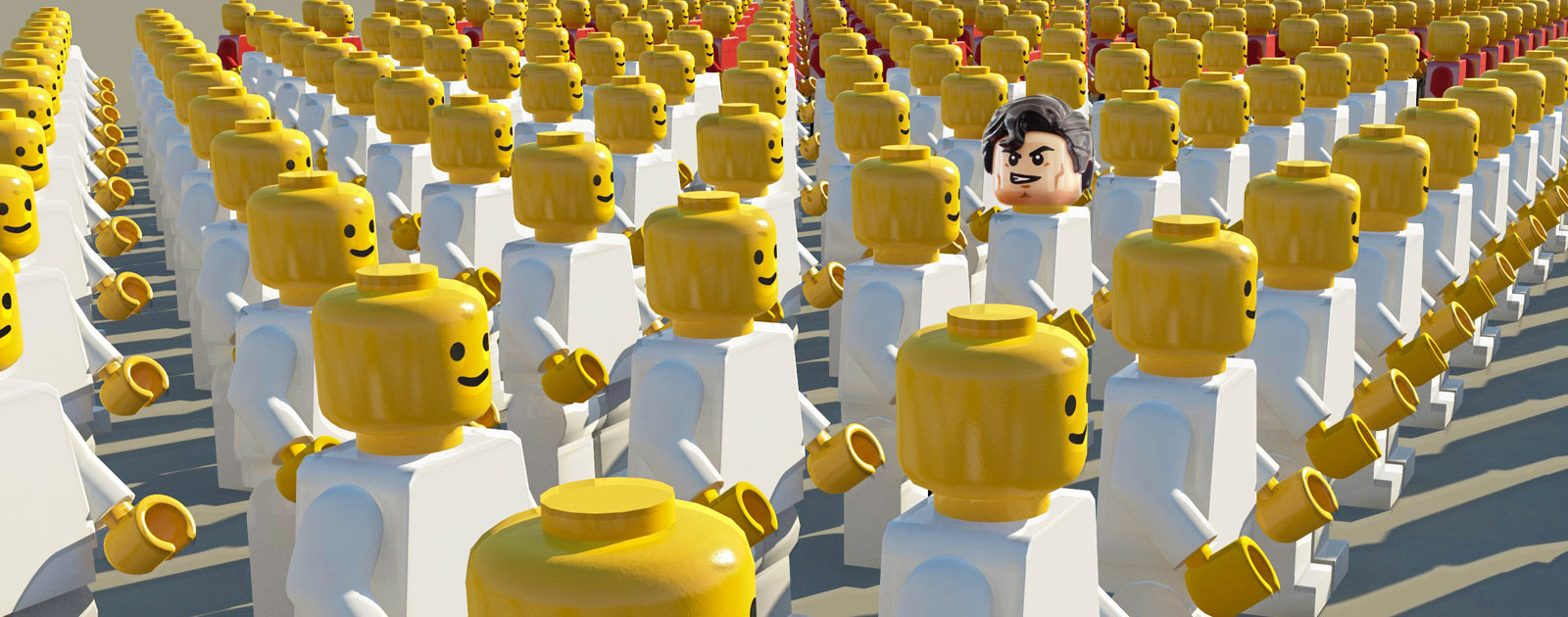
Inspection is a mandatory but nonvalue-adding activity, and our objective is to do as little as possible, provided that we continue to fulfill the customer’s requirements. The zero acceptance number (c = 0) sampling plan requires far less inspection than the corresponding ANSI/ASQ Z1.4 (formerly MIL-STD 105) plan, and becomes viable when the supplier is extremely confident in its level of quality.1
ADVERTISEMENT |
An ANSI/ASQ Z1.4 plan consists of a sample size n, and an acceptance number c. The inspector checks n items, and accepts the lot if c or fewer defects or nonconformances are found. These plans are designed to give (roughly) a 95-percent chance of acceptance at the acceptable quality level (AQL), which is one of the parameters for the plan’s selection.
…
Comments
Consumer Risk or Producer Risk?
Thanks to Mr. Levinson for his paper on C=0 sampling plans. The sample size formula presented in his paper is one of the most useful tools available. I would like to add that recently under AS9138 and ARP9013 the definitions have been standardized for Acceptable Quality Level (AQL), Equal Risk Point (ERP), Lot Tolerance Percent Defective (LTPD), and Rejectable Quality Level (RQL). They are in fact simply different points on the same operating characteristic curve having probability of acceptance 0.90-0.95, 0.50, 0.10, and 0.05 respectively. During world war two, the point-of-view of the consumer (LTPD) was abandoned in favor of the producer's point-of-view (AQL) because, as HR Bellinson stated; 20,000,000 identical items were being procured from more than 50 different suppliers, and a c=0 sampling plan was thought to be unfair to small suppliers; rejecting their product more often than large suppliers product having exactly the same quality level (ASA 105th annual meeting, January 27th 1946.). Of course AQL based sampling plans can have larger sample sizes than c=0 plans in order to "bend" the operating characteristic curve at the AQL point providing a high probability of acceptance, while still holding the same consumer's LTPD point. It is improper to say that a c=0 plan has an AQL because by design, its point-of-view is the consumer, not the producer. This is the reason for the 0.542 probability of acceptance in Mr. Levinson's example with n=15, c=0 for a lot is 4% nonconforming (4.0 AQL). We can not design a set of consistent c=0 sampling plans with the producer's risk in mind. This was the main thrust and birth of AQL based sampling plans.
With the consumer in mind - A sampling plan which is finding the nonconformities most of the time, is doing its intended job and therefore does not need to be tightened. It is only when the economics of the situation dictate that the expense of finding a few nonconformities later outweighs the expense of more inspection that we will tighten inspection.
There are many attribute sampling procedures such as MIL-STD-105, MIL-STD-1916, APR9013, and AS9138 to name a few. Most have differing poin-of-view and it all becomes a little confusing, but thankfully the underlying mathematics remains constant and reveals that it is simply a different color "lipstick" on the same OC Curve.
Why did you use binomial distribution?
Dear Mr. William A Levinson.
I was instroduced Squeglia's c=0 plan at March of 2018. I was wanted to know Squeaglia's calculation logic to define sample size.
I read his book, 5th edition but failed to find out. So I am very thank you for your article. And I have one question on your article.
On Squeaglia's book, He told that he used Hypergeometric distirbution for more exact calculation, but you used only Binomial distribution
to get sample size n. I hope to know why you didn't use Hypergeometric distribution.
The another question is the book or article which Squeaglia wrote his logic and claculations.
On the 5th edition, "Zero Acceptance Number Sampling Plans", I couldn't find out how he calculate sample size n.
If you give me smallest idea on your reply, it will be very appreciated.
I'm looking forward to reading of your reply.
Best regards,
Hong Sung Kim
IQC engineer of display device manufacturer
Republic of Korea (South Korea)
Hi, I noticed your question…
Hi, I noticed your question about using the hypergeometric distribution instead of the binomial distribution, And i hope this helps.
It is generally more reliable to use the hypergeometric distribution when deriving the OC curve, especially because the binomial distribution becomes less accurate when the sample size is large relative to the population size.
As a rule of thumb, if the ratio n/N (sample size to population size) is less than 5%, both distributions can typically be used. However, when n/N exceeds 5% (often the case with smaller populations), the hypergeometric distribution provides more precise results. Therefore, in both scenarios, opting for the hypergeometric distribution is usually the safer choice.
Regards Khibox,
Add new comment