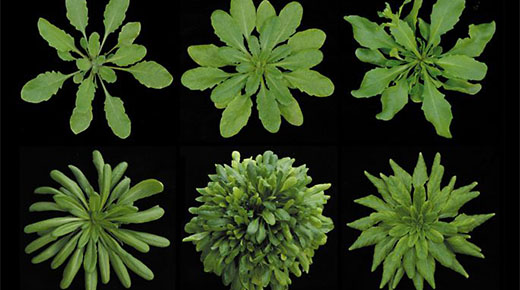
Body
Quality and manufacturing practitioners are most familiar with the effect of variation on product quality, and this is still the focus of the quality management and Six Sigma bodies of knowledge. Other forms of variation are, however, equally important—in terms of their ability to cause what Prussian general Carl von Clausewitz called friction, a form of muda, or waste—in production control and also many service-related activities. This article shows how variation affects the latter as well as the former applications.
…
Want to continue?
Log in or create a FREE account.
By logging in you agree to receive communication from Quality Digest.
Privacy Policy.
Add new comment