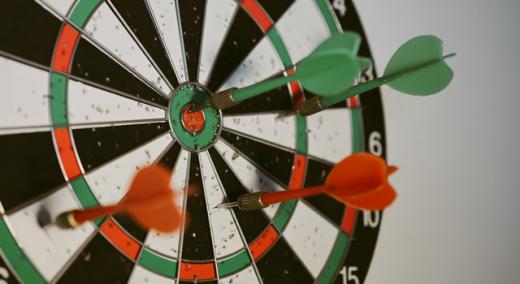
Photo by Afif Ramdhasuma on Unsplash
The difference between common (or random) cause and special (or assignable) cause variation is the foundation of statistical process control (SPC). An SPC chart prevents tampering or overadjustment by assuming that the process is in control, i.e., special or assignable causes are absent unless a point goes outside the control limits. An out-of-control signal is strong evidence that there has been a change in the process mean or variation. An out-of-control signal on an attribute control chart is similarly evidence of an increase in the defect or nonconformance rate.
ADVERTISEMENT |
The question arises, however, whether events like workplace injuries, medical mistakes, hospital-acquired infections, and so on are in fact due to random or common cause variation, even if their rates follow binomial or Poisson distributions. Addison’s disease and syphilis have both been called “the Great Pretender” because their symptoms resemble those of other diseases. Special or assignable cause problems can similarly masquerade as random or common cause if their metrics fit the usual np (number of nonconformances) or c (defect count) control charts.
…
Add new comment