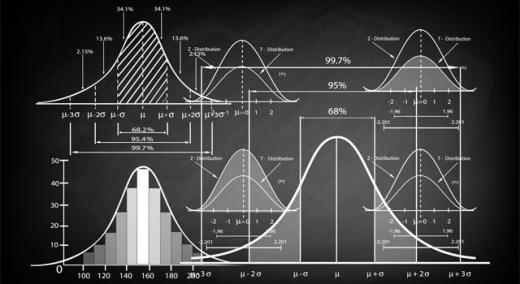
In the past two months we have looked at how three-sigma limits work with skewed data. This column finds the power functions for the probability limits of phase two charts with skewed probability models, and compares the trade-offs made by three-sigma limits with the trade-offs made by the probability limits.
ADVERTISEMENT |
Phase two charts
Ever since 1935, there have been two approaches to finding limits for process behavior charts. There is Walter Shewhart’s approach using fixed-width limits, and there is Egon Pearson’s fixed-coverage approach based on probability models. (For more on these two schools of thought, see “The Normality Myth,” Quality Digest, Sept. 19, 2019.) About the year 2000, some of my fellow statisticians tried to reconcile these two approaches by talking about “phase one and phase two control charts.”
…
Add new comment