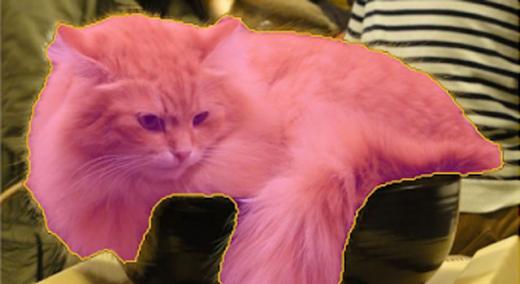
While traditional image processing software relies on task-specific algorithms, deep learning software uses a network to implement user-trained algorithms to recognize good and bad images or regions.
ADVERTISEMENT |
Fortunately, the advent of specialized algorithms and graphical user interface (GUI) tools for training neural networks is making it easier, quicker, and more affordable for manufacturers. What can manufacturers expect from these deep-learning GUI tools, and what’s it like using them?
Training: Creating the deep learning model
Training is the process of “teaching” a deep neural network (DNN) to perform a desired task—such as image classification or converting speech into text—by feeding it data that it can learn from. A DNN makes a prediction about what the data represent. Errors in the prediction are then fed back to the network to update the strength of the connections between the artificial neurons. The more data you feed the DNN, the more it learns, until the DNN is making predictions with a desired level of accuracy.
As an example, consider training a DNN designed to identify an image as being one of three different categories: a person, a car, or a mechanical gear.
…
Add new comment