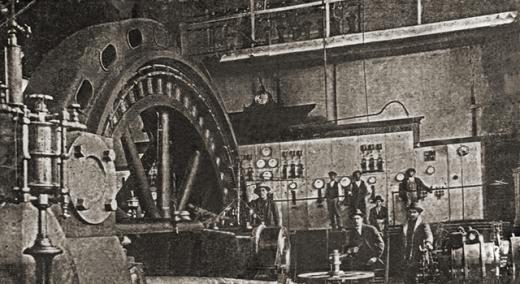
Body
In Parts One and Two we defined the equivalence of instruments in terms of bias and measurement error based on studies using a single standard. Here we look at comparing instruments for differences in bias or differences in measurement error while using multiple standards.
…
Want to continue?
Log in or create a FREE account.
By logging in you agree to receive communication from Quality Digest.
Privacy Policy.
Add new comment