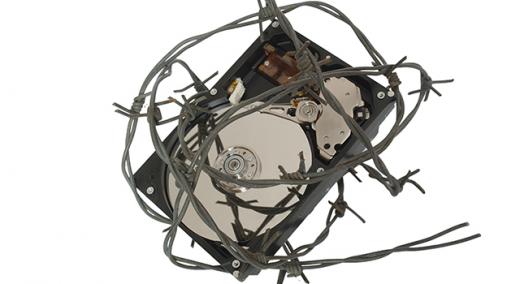
Management requires prediction. However, when making predictions it is easy to torture the data until they surrender and tell you what you expect to hear. Even though this torture may be unintentional, it can keep you from hearing the story the data could tell. This column is about how to avoid torturing your data while making predictions.
ADVERTISEMENT |
A few years ago a correspondent sent me the data for the number of major North Atlantic hurricanes for a 65-year period. Major hurricanes are those that make it up to category 3 or higher. I have updated this data set to include the number of major hurricanes through 2021. The counts of these major hurricanes are shown in a histogram in figure 1. In what follows we shall look at two approaches to using these data to make predictions.
Figure 1: Major North Atlantic hurricanes per year, 1935–2021
…
Comments
Great article!
Great article! Thanks, Dr. Donald J. Wheeler
Send it to the US President
An excellent article again! Given the recent storms across the States maybe send it to the new task force just announced by the President
Control Limits Breakpoints
Dr Wheeler,
Excellent article. As a MBB, I always instruct problem solvers to gather all aspects of the data (who, what, where, when) in order to plot it many ways, and always get the temporal understanding of the data so it can be plotted on a time series plot.
My question is about determining the breakpoints to create new control limits as shown in Figure 5. Is there a certain rule to determine when to create new control limits or is just a trial and error method combined with observing natural breakpoints? On Figure 5, it looks obvious, but I can imagine a lot of cases where it is not so obvious.
Thanks.
Breakpoints
Dr. Wheeler will be able to address your question much better than I, but I have a few thoughts to consider.
- Plot without any breaks and the signals in the behavior chart will give clues about when breaks have happened (use Western Electric or other rules to find signals).
- Make sure signals are breakpoints of trends and not individual or tightly clustered extraordinary causes.
I have done this with many processes and found shifts in the behavior charts. After investigating the circumstances around the breakpoint timeline, I've often found that:
1) intentional changes were made to the process that were assumed to have no effect on this particular metric
2) unintentional changes were made to the process by new operators, maintenance, even the weather (seasonal changes)
Non homogeneous
It is very difficult, if not impossible to get meaningful data in such an area over a long period. Detection has greatly improved, yet.https://www.drroyspencer.com/wp-content/uploads/tornadoes-1954-2018.jpg
If the Operational Definition in "what are we trying to achieve?", is to predict the future, the data is clearly non homogeneous. Predictions are not meaningful.
Add new comment