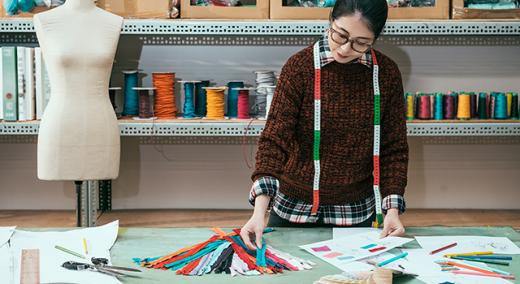
First, let it be known that all charts for count-based data are charts for individual values. Regardless of whether we are working with a count or a rate, we obtain one value per time period and want to plot a point every time we get a value. This need to plot the current data is why the specialty charts for count-based data were developed before a general approach for charting individual values was discovered. The question addressed in this column is when to use the specialty charts with your count-based data.
ADVERTISEMENT |
The first of these specialty charts, the p-chart, was created by Walter Shewhart in 1924. At that time the idea of using the two-point moving range to measure the dispersion of a set of individual values had not yet reached the professional literature. (John von Neumann would introduce the use of successive differences to the mathematical world in 1941, and W. J. Jennett would have the idea of an XmR chart in 1942.)
…
Comments
Use of speciality charts
So I take it that the gist of your message is- if it ain't broke don't try to fix it?
Defectives versus defects
I was taken aback by this article because I had been taught for years that attribute data had to be charted using a chart based on a binomial or Poisson distribution because it was attribute and not variable data. I still do not understand (and your fine article does not reference it) the distinction in the distributional assumption between a defective and a defect and how while different distributions apply (and the formulas for control limits are different) an IX-MR chart can be used for any set of data, attribute or variable and no matter whether it refers to defects or defectives in the count. Would appreciate further understanding as much of what I took as truth has been shaken by your article.
Reply for Ted
Good question. While counts are discrete, this discreteness does not get in the way of the computations until the average count per time peiod falls below 1. So whether we are counting items or events will affect which of the speciality charts we use, it doens not interfere with the empirical computations of the XmR chart. The use of generic, fixed-width, three sigma limits is sufficiently conservative to free us from the necessity of specifying a probability model and computing limits with a fixed probability of exceedance. Shewhart's approach is completely different from the approach of traditional statistical inference. So, as illustrated, when the speciality charts are appropriate, the XmR chart will mimic them. But when they are not appropriate, the XmR chart will still be right.
CUSUM EWMA
Is XmR chart better than a CUSUM or a EWMA chart?
Reply for NCOSTA
CUSUM and EWMA are both slower to detect a change than is the XmR chart.
I have written complete chapters on each of these techniques in my Advanced Topics in SPC textbook.
For more information contact me directly.
XmR vs c, np, p, u
I have found that varying limits in p/u charts cause people to start questioning why the limits vary and how the limits were calculated, which diverts attention from what the chart is telling us.
In the XmR chart, people often wonder why we need a Range chart, but the flat UCL/LCL are not cause for concern. The range chart is easy to explain (differences between points) and the X chart is the actual data.
In the Health Care Data Guide, Provost and Murray argue that p and u charts are more sensitive to special causes, but in my own experience this is more an exception than a rule.
So, as Donald has pointed out before, the XmR chart is the Swiss Army Knife of control charts. It works well and is easy to use. Best of all, people only have to understand one type of chart which makes it easier to train leaders and managers who have never seen an XmR chart before.
Any SPC software will calculate and display these charts effortlessly. Start using the XmR chart. You'll be surprised by how versatile it is.
XmR and Run Charts
Specialty charts are indeed more difficult to utilize and validate. In the many years that I used charts, I found that people understand the XmR chart and Run Charts (median = centerline) much more readily that others. The XmR chart has been descrivbed as the "Swiss Army Knife of Process Behavior Charts by Dr. Wheeler, and I have always found that to be the case.
Add new comment