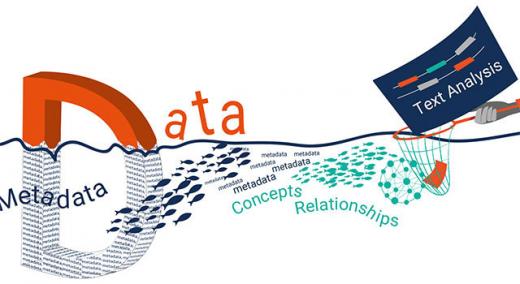
There are four major questions in statistics. These can be listed under the headings of description, probability, inference, and homogeneity. An appreciation of the relationships between these four areas is essential for successful data analysis. This column outlines these relationships and provides a guide for data analyses.
ADVERTISEMENT |
The description question
Given a collection of numbers, what arithmetic functions will summarize the information contained in those numbers in some meaningful way?
To be effective, a descriptive statistic has to make sense—it has to distill some essential characteristic of the data into a value that is both appropriate and understandable. In every case, this distillation takes on the form of some arithmetic operation:
Data + Arithmetic = Statistic
As soon as we have said this, it becomes apparent that the justification for computing any given statistic must come from the nature of the data themselves. The meaning of any statistic depends upon the context for the data, while the appropriateness of any statistic depends on the use we intend to make of that statistic.
…
Comments
Your articles and books are the best source of knowledge!
Dear Donald,
Thank you for your work. Your articles and books are the best source of knowledge!
May God grant you health and long life!
Homogeneous operation
Another brilliant paper, as always.
"When the data happen to be homogeneous, the chart allows you to proceed to analyze your data as if they came from a single universe." What type of such analysis might be useful for a process, while it is operating homogeneously?
Great illustration
This explanatory framework for the different kinds of questions in statistics is especially illuminating. When I try explaining SPC vs traditional statistics to people, they seem to have the best time grasping it when I talk about "methods for describing the bowl of beads based on a handful of beads" versus "the question of whether it's probably still the same bowl of beads as before or not."
Add new comment