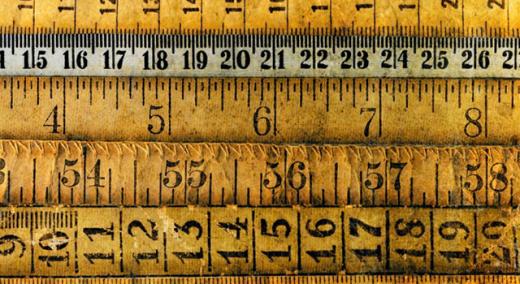
For more than 40 years it has been common to use the precision to tolerance ratio (P/T ratio) to compare the standard deviation of measurement error with the specified tolerance for a particular product. The purpose of this comparison being an assessment of the utility of the measurement process. Here we shall look at what the P/T ratio does and does not do.
ADVERTISEMENT |
The structure of a product measurement
We will need some notation for what follows. So, let the product measurements be denoted by X. These product measurements may be thought of as consisting of two components. The value of the item being measured may be denoted by Y, while the error of the measurement may be denoted by E. Thus:
…
Add new comment