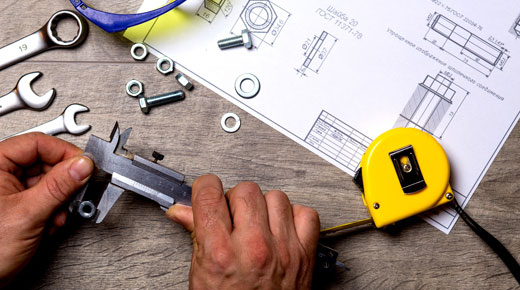
Whenever we make a measurement, we have to decide how many digits to record. Traditional answers for this question are often little more than guesswork glorified by time. And with digital readouts, are all the displayed digits real? This column provides a sound and practical answer to these perennial questions.
There are two uncertainties that affect every measurement. These uncertainties are the round-off and measurement error. The round-off has to do with the uncertainty introduced by the choice of measurement increment. Measurement error is the inherent uncertainty introduced by the measurement system itself. To understand the impact of each of these uncertainties we begin by looking at them separately.
Round-off uncertainty
Assume that the only uncertainty in our measurements is the round-off. Since we only record observations to a finite number of decimal places, there will only be a finite number of possible values for our measurements. These possible values will always end up being some integer multiple of the measurement increment (MI). If we denote our observed value as X, then X will be some multiple of MI.
…
Add new comment