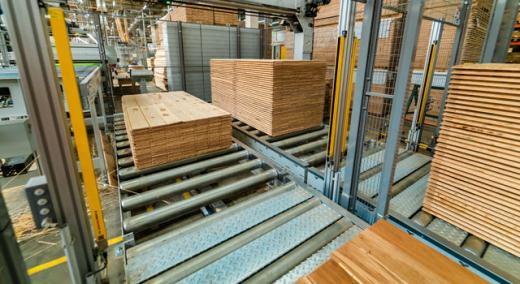
Acceptance sampling uses the observed properties of a sample drawn from a lot or batch to make a decision about whether to accept or reject that lot or batch. Although the textbooks are full of complex descriptions of various acceptance sampling plans, there are some very important aspects of acceptance sampling that are not included in the textbooks.
ADVERTISEMENT |
Extrapolation
In the interest of simplicity, the product that has been measured will be referred to as the “sample,” while the product that hasn’t been measured will be referred to as the “lot.” Every time we attempt to use a sample to characterize the quality of a lot, we will be making an extrapolation from the product that has been measured to the product that hasn’t been measured.
…
Comments
Let's ditch acceptance sampling
Great job, Don. I'm on a campaign to clean up the odds and ends of the quality profession. Acceptance sampling was on my list, but you beat me to it! It frees up another block of misinformation that needs to be replaced with something more useful.
Wonderfully presented.
Thanks for sharing this. The summary is wonderfully worded.
Reprint?
Hello Dr. Wheeler & Quality Digest,
Is this a reprint of the two part article "The Truth About Acceptance Sampling" from Jul and Aug of 2014? Just trying to make sure I am not missing any new bits you might have included. Those two articles from 2014 were incredibly useful to me as I tried to educate my colleagues on acceptance sampling.
Kind regards,
Shrikant Kalegaonkar
Shrikant's Question
This column is a condensed version of that two part article. I do not recall putting anything new in this version. I am glad the earlier version proved useful.
Thank you, Dr. Wheeler
Thank you for clarifying Dr. Wheeler.
Add new comment