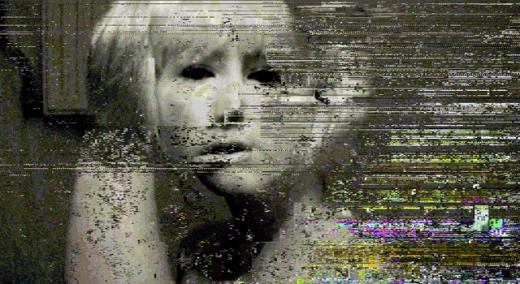
Finding the signal in the noise. "Voided" by Rosa Menkman
Every introductory class in statistics teaches how to obtain a global standard deviation statistic. While this descriptive statistic characterizes the dispersion of the data, it is not the correct value to use when looking for unusual values within the data. Since all of statistical inference is built around the search for unusual values, it is important to understand why we do not use the global standard deviation in this quest.
ADVERTISEMENT |
The descriptive statistics taught in introductory classes are appropriate summaries for homogeneous collections of data. But the real world has many ways of creating non-homogeneous data sets. Some of these involve unknown and unplanned changes in the process or procedure producing the measurements. When these occur we talk about “outliers” and “exceptional values.” Other ways of getting unusual values involve the deliberate manipulation of inputs for the purpose of creating changes in the observed data. Here we talk about detecting “signals” and obtaining “significant results.”
…
Add new comment