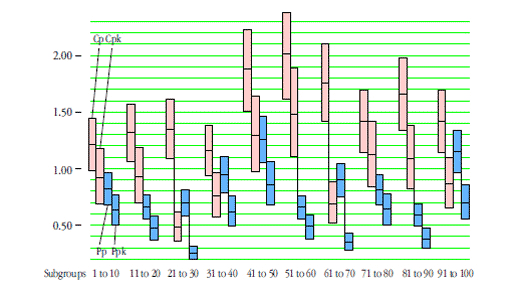
Capability ratios are widely used and sometimes misunderstood. The computer will gladly offer up values of each of the commonly used capability and performance indexes. Yet there is little appreciation of the inherent uncertainty contained in each of these numbers. Here we shall look at how to quantify these uncertainties and how to interpret the ratios.
All capability ratios are statistics. This means that, like all other statistics, capability ratios will vary from computation to computation even when the underlying process does not change. The following examples will illustrate the intrinsic and extrinsic components of this variation.
A predictable process
Figure 1 shows 100 subgroup averages and ranges for subgroups of size 5 based on 500 values obtained from a bead board (or quincunx) while no changes were made in the configuration of the bead board. When operated in this manner the bead board serves as a prototype for a predictable process. The grand average is 10.084 and the average range is 4.11.
Figure 1: Average and range chart for figure 10 data
…
Add new comment