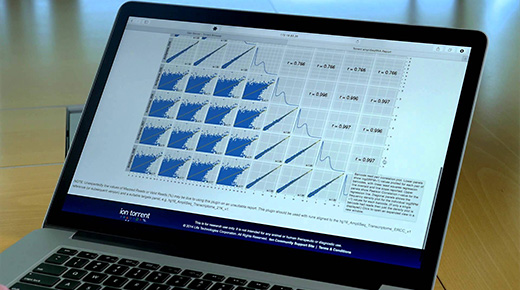
Sometimes we use a chart for individual values and a moving range (an XmR chart) to assess the homogeneity of a finite data set. Since this is an "off-label" use for the XmR chart, we first consider the drawbacks associated with using a sequential technique as a one-time test, and then present an adaptation of the X chart (the analysis of individual values or ANOX) that functions like other one-time statistical tests.
The XmR chart
Like all process behavior charts, the XmR chart was designed for the sequential analysis of a continuing stream of observational data. Here the data will generally represent one condition, and the purpose of the chart is to identify unplanned changes in the underlying process. After the baseline period, where we compute the limits, we extend the limits forward and continue to add data to the chart. Each time we add an additional point to the chart we are performing an act of analysis. Each of these analyses asks if the current value is consistent with the baseline period. And, as with all sequential procedures, we want to perform each of these acts of analyses in a conservative manner in order to reduce the overall risk of a false alarm.
…
Add new comment