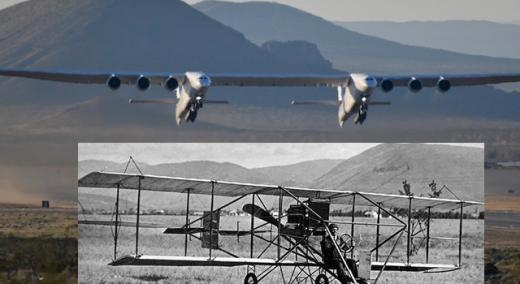
During the past three months James Beagle and I presented columns that made extensive use of analysis of means techniques. Since these techniques may be new to some, this column explains when to use each technique and where to find tables of the appropriate scaling factors.
ADVERTISEMENT |
In 1967, Ellis R. Ott published his analysis of means technique (ANOM) for comparing treatment averages with their grand average. This technique is a generalized version of the average and range chart. However, the assumption that allows this generalization also imposes a restriction of where this technique can be used. The generalization allows us to compute limits with a fixed overall alpha level (the user-specified risk of a false alarm). The restriction is that we can only use ANOM for the one-time analysis of a finite amount data (such as occurs in experimental studies).
…
Add new comment