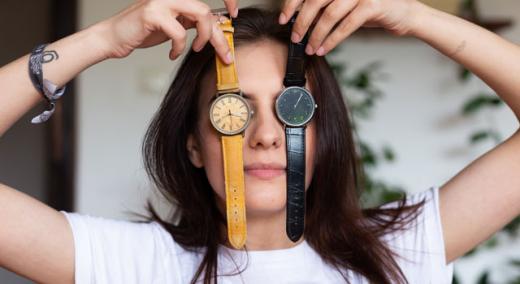
A quick Google search returns many instances of the saying, “A man with a watch knows what time it is. A man with two watches is never sure.” The doubt implied by this saying extends to manufacturing plants: If you measure a product on two (supposedly identical) devices, and one measurement is in specification and the other out of specification, which is right?
ADVERTISEMENT |
The aforementioned doubt also extends to healthcare, where measurement data abound. As part of the management of asthma, I measure my peak expiratory flow rate (discussed below), and I now have two handheld peak flow meter devices. Are the two devices similar or dissimilar? How would I know? To see how I investigated this, and to see the outcome, read on. A postscript is included for those wanting to dig a bit deeper.
Background
In 2015, I was diagnosed with asthma, a chronic condition where the airways in the lungs can narrow and swell, making breathing more difficult. The worst of it occurred at my in-laws, where I experienced wheezing and had difficulty breathing. The cause? The family cat!
…
Add new comment