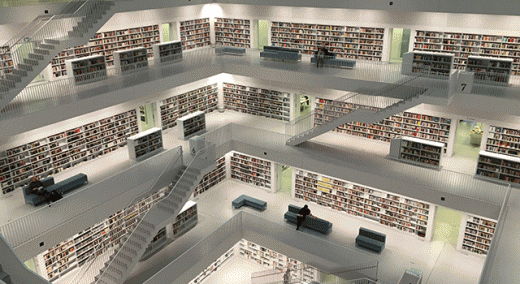
In our ongoing series of CIMdata articles on engineering.com, we’ve focused primarily on the digital (aka “virtual”) and physical aspects of digital transformation. Our discussion of these digital and physical elements has centered on what needs to be done with (and to) information as competitive and innovative new products, systems, services, and assets are developed, produced, and supported.
ADVERTISEMENT |
In the past two years, these articles have addressed almost everything being done to create and manage data and information throughout product life cycles within business units, and by suppliers and contractors who keep the extended enterprise profitable. In this article on data governance, however, I’m changing gears to address management, policies, procedures, and even “people” issues related to information handling to ensure it’s always right for use.
I want to shift attention away from computer monitors, apps, systems, and solutions to instead look at how best to keep all the moving parts in the digital enterprise from running off the rails. I’ll address the need for:
…
Add new comment