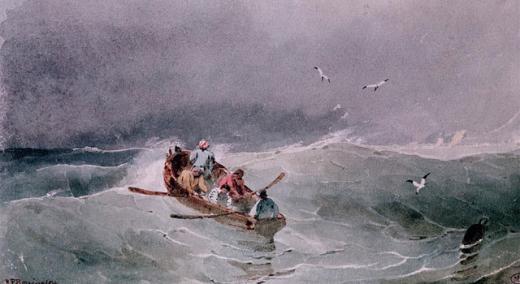
Students are told that they need to check their data for normality before doing virtually any data analysis. And today’s software encourages this by automatically providing normal probability plots and lack-of-fit statistics as part of the output. So it’s not surprising that many think this is the first step in data analysis.
ADVERTISEMENT |
The practice of “checking for normality” has become so widespread that I have even found it listed as a prerequisite for using a distribution-free nonparametric technique! Yet there is little consensus about what to do if your data are found to “not be normally distributed.” If you switch to some other analysis, you are likely to find it too is hidden behind the “check for normality” obstacle. So you are left needing to customize your analysis by fitting some probability model to your data before you can proceed—and this opens the door to all kinds of complexities.
The histogram in Figure 1 represents 20 days of production for one product. It is clearly not going to pass any test for normality. But is there some other probability model that might be used?
…
Comments
Control Chart Origin
Even as a non-statistian, it seems clear that Shewhart did not have a distribution requirement for using process behavior charts. So why do highly educated folks still refuse to accept Shewhart's rational? Any thoughts on that? I just don't get it.
Rich
Good question
Thank you, struggle with the same question. Of course, the first hard question is whether you are mad or if everyone else is mad (statistics professors included). If the conclusion is that the people who likely have a higher IQ than you (the statistics professors) are wrong, then the question is why they are wrong.
Maybe it is because we are all mostly just bald apes trying to do our best in the hierarchies, and that intelligent people are just better at arithmetic. The ability to ask the basic questions, however, might be something that is spread much more scarcely amongst the species.
Or maybe there are other, more specific answers that lend themselves better to constructive criticism of classical SPC. It would be great if someone dared ask text-book authors like Douglas Montgomery how they respond to the criticism. That would require people to be able to ask the basic questions, and not just be good at arithmetic. As mentioned, the answer on that is not obvious.
Montgomery.
My paper here exposes some of Montgomery's nonsense:
https://www.linkedin.com/pulse/control-charts-keep-simple-dr-tony-burns/
He did not respond.
Dr Wheeler's book "Normality and the Process Behavior Chart" proves Dr Shewhart's assertion by testing 1143 different distributions. The book is an essential read.
Highly educated folks are human too
Thanks to Donald J. Wheeler I have been learning much about common statistical misconceptions. Where do they come from? Consider reading "Statistics Done Wrong: The Woefully Complete Guide" by Alex Reinhart. At the end he ponders the problem of misonceptions, hyopthesizing that the standard lecture teaching model is to blame. As I recall, his point is that students come to the class with prior knowledge -- misconceptions -- and in the class those misconceptions don't get revealed or they don't get pummeled into non-existence with the lecture model. He also said, probably paraphrasing, "Misonceptions are like cock roaches. They are everywhere, even when you least expect them. And they are impervious to nuclear weapons." These students go on to become pharmaceutical researchers, doctors, scientists, etc. I extend his concept to this: humans become rather enamored in what they believe. When facts are presented that call into question those beliefs, the beliefs often live on, in defiance of the facts. Yes, even engineering, math, science and technology specialists are subject to this human trait.
Why on transformation?
There are two reasons I can think of off-hand for transformation of data (and I agree with Wheeler on the underlying assumption of homogeneity is theoretically correct but pragmatically not in play)
1. Need to understand the distribution of the product being sent in the truck
2. Modelling data that realistic prediction intervals are required
Other than that, transformation should never be discussed in my opinion. Unfortunately, it is propagated all of the time in training because we confuse these two specific needs with all other applications. As Wheeler rightfully argues, if we have unusual values finding a distribution making them IN control defeats the whole purpose. If a probability plot fails the A-D test but shows that it is merely an outlier, then the data IS close to normal. The failure is co-mingling of purposes and Wheeler is correct that concerns over normality don’t apply here until we have a “stable” process. Then limits have a basis in probability. I was fortunate I spent 15 years in an industry that nothing was normal. We solved lots of problems never transforming the data.
Who do you think is right, you or Shuhart? :)
Thank you Dr. Donald J. Wheeler.
I study all your articles with great interest. There is always a lot of useful information in your articles for those who work with real processes. And a subtle sense of humor "who do you think is right, you or Shuhart?" in your articles adds a special relevance to the articles.
Kind Regards,
Sergey Grigoryev
Scientific Director at Center AQT (Advanced Quality Tools)
DEMING.PRO
Great article, as always,
Great article, as always, Don.
You say: "The practice of “checking for normality” has become so widespread." The reason is that keeping it simple doesn't make money for consultants, nor does it help the sale of irrelevant and unnecessary statistical software. In most circumstances, Process Behavior Charts can be drawn manually, and in doing so, gives better insight into what's happening.
Keeping it simple and sticking to the fundamentals, is what clients need to learn. Clients need to learn to avoid buying into the latest fads, farce and fraud.
Everyone should purchase your brilliant book "Normality and the Process Behavior Chart". It is very easy reading and most entertaining.
Tony
How about Central Limit Theorem?
I agree that don't need checking for normality of data sample, but it due to application of Central Limit Theorem (CTM). With CTM, whatever distribution doesn't matter. Is it any point conflict with this topic? To me, agree with this topic, control chart is needed for assignable causes, but nothing involving with data distribution. Am I wrong? Anyway, if an experiment with small scale (10 samples, limited by resource for example), control chart also has quite limited meaning, is it right?
Add new comment