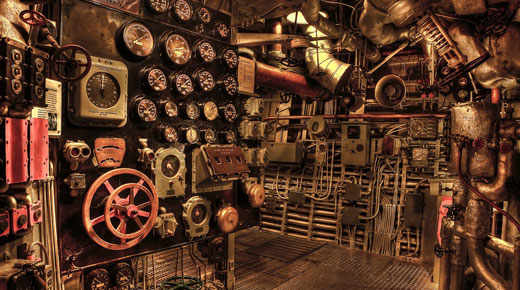
Everybody wants to design and conduct a great experiment! To find enlightenment by the discovery of the big red X and perhaps a few smaller pink x’s along the way. Thoughtful selection of the best experiment factors, the right levels, the most efficient design, the best plan for randomization, and creative ways to quantify the response variable consume our thoughts and imagination. The list of considerations and trade-offs is quite impressive. Then, finally, after optimizing all these considerations, and successfully running the experiment, and then performing the analysis... there is the question of “statistical significance.” Can we claim victory and success?
ADVERTISEMENT |
The answer lies in-part on the critical value provided by a table of critical values—or by a computer program. If our calculated test statistic exceeds the critical value, we will reject the null hypothesis and claim there is a difference among the treatment averages. If our calculated test statistic does not exceed the critical value, we will fail to reject the null hypothesis. This is the moment of truth at the end of all our hard work. This is a moment of anticipation and excitement.
…
Add new comment